Unveiling the UK Scam Tapestry: A Comprehensive Data Analysis
Unveiling the UK Scam Tapestry: A Comprehensive Data Analysis
Table of Contents
Research Findings and Analysis
Evaluating the SPAM Email Detection System.
Evolution of Fraudulent Offences and Computer Misuse with Predictions
An Insight into the Personal and household characteristics of who were victims of fraud.
Prevention and Security Measures.
Critical Analysis and Conclusion.
Summary of Key Findings and Theoretical Contributions.
Implications for Future Research.
References
Abstract
Kavindu Randula Jayarathna Lelwala Hewage has conducted this research on the rising issue of digital scams in the United Kingdom, a phenomenon that significantly affects the country's economic stability of the country as well as the trustworthiness of online services This research is investigating the complex background of fraudulent activities by assessing the temporal and geographical patterns, demographic characteristics, and individual characteristics of the reported scams. This project is to advance understanding and develop effective strategies to mitigate these fraudulent activities through the use of predictive modelling. In addition, intend to conduct an exhaustive analysis of every category of fraudulent activity, including computer misuse. These consist of details such as the attributes of the victims, the behaviours of the offenders, and the methods employed by law enforcement to detect fraudulent activities. The introduction of ethical aspects, following existing legal regulations, and applying countermeasures to identify mistakes in data and the complexity of fraudulent methods is the most important parts of the research. The project also had the web-based scam detection model that was installed in order to identify the scam emails.
The project aims to enhance the UK's financial ecosystem's ability to withstand scams by strengthening social resilience and promoting collaboration across sectors. The primary objective of this undertaking is to protect individuals and businesses against financial exploitation and to reinstate trustworthiness in the digital environment. This study makes considerable progress in addressing the problem of fraudulent activities in the digital era by conducting a thorough examination and utilizing data analytics and machine learning.
Introduction
Foundational Insights
Digital technology in the UK has gone through a development stage and scams have become more complex. This brings about the social and psychological issues of welfare of the individual and financial market. Digital media proliferation and presence have led to an increase in the number of all types of online fraud, as well, including phishing, retail fraud online, investment fraud, and romance fraud. They can be the source of substantial burden for a country’s economy and integrity of online services due to the fact that consumers lose their confidence. The fact that educational programs have to be made to ensure that the out of school population of older adults can develop computer literacy skills shows that education should not be limited to the school environment (Lee, 2018). Criminal activity and online fraud in the UK in fact gained momentum with the lockdown measures enacted against COVID-19 as the digital environment became the new space for virtual criminal activity (Buil-Gil et al., 2021a). Online romance scams have become the most common form of scams in which the criminals develop complex relationship patterns in the end that usually results in serious psychological and financial consequences to their victims.(Coluccia et al., 2020a)
Crypto exchange frauds that have caused massive financial losses hence necessitate drastic and fast preventive measures (Xia et al., 2020). Digital crimes could be successfully handled with the help of international co-operation and legal framework harmonization; the result of this approach is founded on legal comparison of two countries. Moreover, the people are now facing the ever-increasing technological development that besides fuelling fraudulent activities also reveals the digital gap that in the consequence deepens social inequality and digital poverty (Faith, Hernandez and Beecher, 2018).
Research questions
- What is the specific time and location trends related to documented fraudulent activities in the United Kingdom?
- What is the role of demographic vulnerabilities in the frequency of various scam types?
- Which machine learning techniques are helpful in predicting and categorising different types of fraud, such as phishing, investment fraud, and online retail scams?
- Which ethical considerations and legal compliance procedures should be integrated into the construction of a predictive model for identifying and preventing scams?
Research Objectives
- Examine the time-based and location-based trends, demographic susceptibilities, and unique
- characteristics of documented fraudulent activities.
- Create a sophisticated predictive model utilising machine learning methods to detect and
- classify different forms of fraudulent activities.
- Suggest a set of thorough preventive measures, taking into account the intricate nature of scam techniques and ethical factors.
- Promote inter-sector cooperation to implement a comprehensive strategy to address fraud in the United Kingdom.
Scope of Study
This project covers the whole process of a research which is the studying and understanding of the current scamming trends in the UK. It also highlights the creation of a web-based application that serves as a scam detection device. The framework of this programme rests on the fact that there is a need to understand the dynamic nature of cyber scam; where there has been a progressive increase of complexity and hardship, especially in the context of the digital transformation of society and the world economy.
1. Contemporary Analysis of in the UK:
The initial step of the project is an in-depth research of current digital scams phenomena within the UK. This study is based on the recent research that shows that cybercrimes online including phishing and scams have increased the number of people who are victims in the UK, offenders and the national enforcement agencies as well as the data about how they react to these incidences and how they are affected by the COVID-19 pandemic and the subsequent digitalization of people's activities for both professional and personal use. To illustrate, (Coluccia et al., 2020b) reports that cybercrime incidents were greater during lockdown periods, signifying the importance of controlling this issue.
The scope of the investigation centres on the complex characteristics of contemporary fraudulent schemes, their repetitive structures, and the societal and technological elements that enable their occurrence objectives are two-fold: to enhance cyber security and safeguard at-risk communities, and to provide valuable insights to shape policy frameworks. In order to accomplish these goals, suggest an innovative content analysis model for the detection of scams, providing a hopeful resolution in the battle against deceit. (Howell et al., 2022)
The consequences of fraudulent schemes are significant. Victims experience monetary damages and emotional turmoil, while the overall societal expense is significant. The research is warranted by the recent surge in cyber-dependent crimes and online fraud, as evidenced by the rise in incidents during the COVID-19 pandemic. During this time, there was a notable transition of criminal behaviour to the internet, which intensified the dangers linked to digital finance and transactions. The COVID-19 pandemic worsened this issue, leading criminals to shift their activities to the online domain, resulting in a significant increase in cybercrime and online fraud. The increasing prevalence of online shopping scams, auction frauds, and social media hacking illustrates a concerning depiction of a developing criminal environment that specifically preys on unsuspecting individuals.
2. Development of a Scam Detection Platform
The project entitled "Data-Driven Scam Prevention" will now focus on creating the web-based application to detect and prevent frauds, using the knowledge gained from the analysis phase. This application, tacitly called "SCAM Email Detention," will use powerful algorithms and artificial intelligence to detect scams as they occur. Consider all six data models before starting the modelling to decide the model type or the typologies of scams found in the analysis step will help to develop an efficient design, taking into account that the scams are captured from the beginning. Credibility assessment will be one of the functions of the application. It will have user-friendly interfaces that will allow individual consumers and businesses to access features that help them ascertain the authenticity of online interactions and transactions.
The Elaboration Likelihood Model (ELM) theoretical framework elucidates the emotional impact in question. ELM presents two distinct processing pathways: a central route, which involves deep cognitive analysis, and a peripheral route, which relies on external cues. Emotions can influence to take the peripheral route, resulting in impulsive decisions based on superficial characteristics rather than careful analysis. For instance, a favourable emotional state could cause to disregard any discrepancies in a reward-oriented email because of the anticipation of potential benefits.(Norris and Brookes, 2021) Gaining a comprehensive understanding of these mechanisms is essential for effectively reducing online fraud. Educational campaigns have the potential to enhance awareness regarding emotional manipulation tactics and promote more profound cognitive engagement with online messages. Moreover, the ability to recognise individuals who are undergoing negative emotions could facilitate the implementation of focused interventions that provide assistance and deter impulsive actions.
This research is logically structured by using chapters that together are a solid and complete knowledge base about frauds. Begin by providing an introductory background to the historical context and the focus of research. Then follow with an in-depth review of literature examining the evolution of scams, different forms the scams take, and current strategies for detection and prevention. The main event of study is presented in the methodology part, which explains research design, data collection processes and the development of model. The analysis section demonstrates that the model is indeed capable of identifying deceptive content in different data sets, which shows it efficacy. Through a thorough evaluation of the findings, not only testify their importance but also shed light on the steps for future studies. Through the fusion of historical understanding with the latest technological techniques, this study seeks to provide tools for both individuals and organizations in their struggle against fraud, which is a dynamic entity, with the ultimate goal of creating a more secure digital environment.
Literature Review
The Evolution of Scams
The evolution of scams mirrors the larger story of human advancement. Primitive methods of deceit relied on fundamental strategies such as theft and manipulation of trust. Nevertheless, technology has created a favourable environment for the emergence of innovative types of fraudsters. The comprehensive chronicle of the 1963 Great Train Robbery showcases the audacious and well-coordinated character of criminal enterprises, underscoring the capacity for substantial economic and societal consequences. These historical events serve as a reminder of the persistent danger presented by scams and the need for alert and creative countermeasures. (British Transport Police, 1963) Although historical events provide valuable insights, it is important to recognize that nostalgia is not useful in addressing current threats.
The psychological dimension of digital scams for example online romance scams, is an illustration of the complex relationship between social dynamics and the weak spots of people that seek love online. the film gets into the psychological profiles of the victim and the hustler, revealing the emotional and financial havoc that is caused by such scams (Coluccia et al., 2012). This body of work indicates that the scams of the digital era are not only enhanced by their high-tech features but also by their psychological manipulation.
Being the UK, the legal and legislative systems have been changing and evolving in the face of digital scam, however, the challenges are still there in terms of combating these sorts of crimes. According to Bokovnya, the article examines legislation on both sides of the Atlantic, implying the importance of international cooperation and legal harmonization for the cross-border nature of digital fraud(Bokovnya, andegishev,2019).
Current Overview in the UK
The incidence of scams, which involve deceitful tactics aimed at manipulating individuals for personal benefit, has significantly increased in the UK, posing difficulties that extend beyond just financial losses. These actions elicit emotional anguish and erode trust within the community. Comprehensive research of academic literature is crucial for comprehending the intricacies of scams, which include examining major inquiries, disputed aspects, and theoretical frameworks that are peculiar to the United Kingdom. One of the main difficulties is assessing the extent to which scams are widespread, which is influenced by their varied characteristics and the harm they do to victims. The rise of online romance scams in the UK highlights the changing nature of fraudulent activities, with about 230,000 British individuals being targeted by these schemes as of 2012 (Whitty and Buchanan, 2012)The reference is from a study conducted by Whitty and Buchanan in 2012. Moreover, scams have significant health consequences for elderly adults, illustrating the connection between financial fraud and psychological welfare, as demonstrated by the detrimental effects on mental health, self-worth, and social interactions.(Eost-Telling et al., 2019)
According to the Annual Fraud Report of 2022, fraudulent losses in the United Kingdom amounted to £1.2 billion in 2022, including both authorized and unauthorised transactions. This represents an 8% decline in comparison to the preceding year. There was a 4% decline in the number of confirmed cases of fraud, which totalled 2,988,705 incidents. More precisely, the number of losses attributable to unauthorised financial fraud (including but not limited to cheque fraud, remote banking, and payment card fraud) decreased marginally to £726.9 million. By implementing effective fraud prevention measures, financial institutions successfully prevented around £1.2 billion in unauthorised fraudulent attempts, resulting in a prevention rate of 61.5p per £1 attempted (UK Finance, 2022). Moreover, according to UK Finance (2022), 207,372 authorised push payment (APP) schemes were documented in 2022, resulting in £485.2 million in losses. This represents a decline from the £583.2 million lost in the previous year. As a result of the post-pandemic reshaping of the fraud landscape, which saw a decline in some forms of fraud and an increase in others, fraud statistics fluctuate. Fraudsters persistently utilise a multitude of platforms, such as search engines and social media, to manipulate victims into disclosing sensitive personal and financial data. Particularly in schemes involving the theft of personal information, social engineering continues to be the most prevalent method for carrying out both unauthorised and authorised fraud, which substantially increases losses(UK Finance, 2022).
An authorized push payment (APP) scam incident occurred in the United Kingdom in 2018, resulting in significant financial losses for personal and business banking clients amounting to £354 million. Recovery efforts yielded a meagre £83 million.(UK Finance, 2019) These fraudulent activities, in which victims transfer funds to perpetrators without their knowledge, emphasise the critical necessity for enhanced protections against these deceitful strategies. Regulatory bodies in the United Kingdom, such as the Financial Conduct Authority (FCA) and the Payment Systems Regulator (PSR), have expressed their support for the Contingent Reimbursement Model Code (CRM Code). This initiative, which will go into effect on May 28, 2019, requires Payment Service Providers (PSPs) to reimburse victims under certain conditions in an effort to cover more than 85 percent of UK payments. Although the CRM Code has good intentions, its efficacy is impeded by its discretionary nature and the stringent reimbursement criteria. As a result, continuous evaluation and a broader application are required to encompass a variety of fraudulent activities and payment services.(Dahlgreen, 2023) These occurrences underscore the critical necessity for technological advancement and regulatory change in order to confront the complex obstacles presented by financial scam risks. They further emphasise the significance of ongoing evaluation, adjustment, and wider adoption of preventive measures.
Ethical Considerations
The emergence of online platforms has brought about substantial changes in the annals of scams in the United Kingdom. According to the 2019 Crime Survey for England and Wales, there were an estimated 3.8 million cases of fraud, which accounted for approximately one-third of all criminal activities. This transition from traditional, physical crimes to cybercrime has resulted in the formation of a more decentralised criminal network that employs digital tools to communicate securely and anonymously. Notwithstanding the progressions made in the field of cybersecurity, a significant number of cybercrimes continue to go undetected, thereby presenting an ongoing and ever-changing menace(Spam, scam, scam - ProQuest). The study "Cybercrime and Shifts in Opportunities During COVID-19" identified a surge in cybercrime incidents, specifically online fraud and hacking, as a consequence of the pandemic. This finding underscores the manner in which the pandemic has facilitated the transition of criminal opportunities from physical to digital environments. This underscores the necessity for enhanced cybersecurity protocols and consciousness in order to confront the ever-changing realm of cyber threats (Buil-Gil et al., 2020) This analogy demonstrates the dynamic progression of cybercrime within the United Kingdom, emphasising the criticality of adjusting to emerging risks and fortifying preventive measures.
Society's dependence on social media has become an indispensable component of everyday existence due to the exponential growth of technology. The platforms' unrestricted access and user-friendly design facilitate seamless engagement with digital content for a broad spectrum of individuals. Nevertheless, this ease of access also facilitates the widespread dissemination of deceptive advertisements and fraudulent schemes. Scammers' ability to target specific demographics, such as individuals with particular interests and age groups, with sophisticated social media advertising tools that were originally designed to assist businesses in promoting their products and services is a particularly concerning aspect.(Al-Rousan et al., 2020) By taking this specific approach, con artists are able to establish direct communication with potential victims, thereby expanding the scope and efficacy of their fraudulent activities. The ethical ramifications associated with the improper utilisation of advertising technology emphasise the necessity for stricter regulatory policies and increased public consciousness in order to effectively address online scams.(Al-Rousan et al., 2020)
The ethical implications associated with fraudulent advertisements in the United Kingdom, as exemplified by incidents such as JWT India and Ford Figo, underscore the discourse on producing advertisements for the sole purpose of securing awards without obtaining client consent. These practices, although more widespread on a global scale, give rise to substantial ethical concerns in the advertising sector of the United Kingdom with regard to veracity and ethical conduct. The persistence of the temptation to use fraudulent advertisements to bolster an agency's reputation is noteworthy, notwithstanding the rigorous ethical and regulatory standards that may discourage their prevalence in the United Kingdom.(Do scam ads, ProQuest) In a competitive market environment, creative leaders in the United Kingdom place a premium on genuine client projects, delivering authentic strategic creative solutions while preserving client confidence. This position signifies a more extensive dedication of the advertising industry to ethical advertising methods, placing significant emphasis on the virtues of genuineness and ethical accountability as means to cultivate confidence and integrity within the sector.
Impact of COVID-19 on the Prevalence of Online Scams
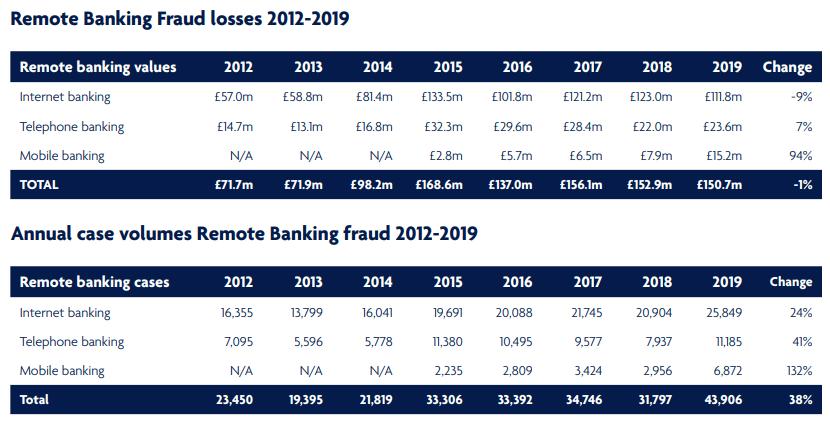
Source: (UK Finance, 2020)
In the context of scams, Amidst the COVID-19 pandemic, there was a notable surge in online fraudulent activities within the United Kingdom. Authorised push payment (APP) scams constituted the most prominent form of such fraudulent activities, resulting in a 5% escalation in financial losses totalling £479 million.(UK Finance, 2020) The banking industry was able to repatriate £206.9 million to the victims despite these obstacles. Furthermore, the industry successfully thwarted a multitude of unauthorised fraudulent endeavours, resulting in an overall depreciation of £783.8 million. During this time period, there was a notable surge in impersonation scams, which almost doubled in number. Investment and romance scams also experienced substantial expansion, underscoring the evolving tactics of fraudsters in the midst of the pandemic (Buil-Gil et al., 2021b).
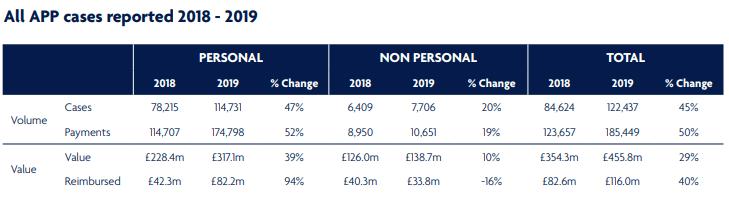
Source: (UK Finance, 2020)
UK Finance has been an outspoken proponent of legislative measures mandating the removal of fraudulent content from online platforms without delay. The significant increase of 22% in reported APP fraud cases emphasises the critical nature of implementing stronger cybersecurity protocols in order to counter the escalating incidence of cyber-financial crimes. Collaboration between law enforcement and the banking industry has resulted in substantial apprehensions and prevented further financial losses, showcasing a proactive stance in combating cybercrime amidst the pandemic(Kemp et al., 2021). Furthermore, fraudulent activities and errors caused the UK's COVID-19 emergency loan schemes to incur losses of up to £16 billion, in addition to the £129 billion in loans disbursed during the pandemic. Due to the expeditious implementation of these initiatives, they became susceptible to substantial fraud risks; this emphasises the necessity for enhanced fund recovery endeavours and the formation of a "taxpayer protection taskforce." The government foresees the classification of irrecoverable loans amounting to £21 billion as losses(Josyula, Vishnubhotla and Onyando, 2023).
Advancements in Fraud Detection Technologies
This underscores the difficulties and demands for increased accountability and readiness in anticipation of forthcoming emergencies. To address these challenges, scholars have put forth inventive technological remedies. In order to facilitate the reimbursement process for individuals who have fallen victim to fraud, Abadi and Murdoch (2023) devised the "Payment with Dispute Resolution" (PwDR) protocol, which ensures both confidentiality and effectiveness. This methodology presents a potentially effective resolution for augmenting the safeguarding of victims and the clarity of banking operations(Abadi, Murdoch and Zacharias, 2023). Bandyopadhyay (2020) showcased the efficacy of recurrent neural networks in identifying fraudulent transactions amidst the pandemic, attaining a remarkable 99.87% accuracy rate. The aforementioned approach underscores the capacity of deep learning technologies to detect and avert fraudulent behaviours (Bandyopadhyay, 2020). Ma, Dhot, and Raza (2023) investigated the application of artificial intelligence to the management of APP scams, thereby demonstrating the potential and obstacles associated with employing AI to combat cybercrime. The insights they offer regarding the implementation of AI technologies for the prevention and management of fraud are of great value(Ma, Dhot and Raza, 2023). By utilising advanced technologies to improve fraud detection and prevention strategies, these studies underscore the criticality of protecting the financial security of businesses and individuals throughout and after the pandemic era.
Application of Artificial Intelligence in Cybersecurity
The novel anti-phishing training method (APTIPWD) enhances users' capacity to differentiate between phishing and legitimate websites, surpassing the effectiveness of conventional email-based anti-phishing tips. This method provides users with real-time education, promptly correcting their errors to improve their ability to identify phishing threats. The research, carried out in 2009, demonstrated that the APTIPWD method is superior in assisting users to accurately identify phishing websites. The study entailed conducting an experiment where participants were divided into groups in order to compare the efficacy of the new approach with the traditional email tip method. The findings demonstrated a notable and favourable impact of the APTIPWD technique, as it led to improved accuracy in distinguishing between authentic and fraudulent websites by users. The paper promotes the implementation of ongoing user training in identifying phishing attempts, in addition to technical measures, to reduce the occurrence of phishing scams, specifically in the domains of online banking and e-commerce. This study emphasises the significance of prompt and situational training interventions to enhance cybersecurity awareness among internet users.(Alnajim and Munro, 2009) The surge in online transactions has resulted in an escalation of credit card fraud, necessitating the utilisation of machine learning (ML) methodologies for detection. This study employs machine learning algorithms, specifically logistic regression and support vector machines, to examine a dataset of European credit card transactions and detect instances of fraudulent behaviour. The study entails partitioning the data into separate training and testing sets, implementing machine learning models, and assessing their efficacy by measuring accuracy and precision.(Bains et al., 2021)
This initiative is a joint endeavour by Microsoft Research and the Microsoft Digital Crimes Unit aimed at tackling the widespread problem of Technical Support Scams through the use of malicious webpages and advertisements. By utilising cloud-based artificial intelligence technologies, a comprehensive system has been developed to identify and prevent fraudulent activities on a worldwide level. This system is crucial in law enforcement efforts, specifically aiding the Federal Trade Commission (FTC) in carrying out major crackdowns on scam networks in 2016. Between 2016 and 2018, the adoption of this approach resulted in a 5% decrease in the number of consumers being targeted by these fraudulent activities. The pipeline efficiently detects 500-600 new instances of scams on a daily basis by utilising machine learning and distributed computing for immediate scam identification. The data obtained from this procedure is systematically arranged into a graph to facilitate meticulous forensic examination. This allows investigators to closely monitor and comprehend the evolving strategies employed by scam operations. This study underscores the efficacy of incorporating AI technologies to combat Technical Support Scams and underscores the crucial role of collaboration between tech corporations and law enforcement agencies in reducing online fraud. (Larson et al., 2018)
Although individual awareness campaigns are important for prevention, a comprehensive strategy is required to effectively combat scams. Policy interventions, such as implementing comprehensive regulatory frameworks and fostering collaboration between financial institutions and law enforcement agencies, are crucial in order to effectively disrupt the operations of scammers and ensure their accountability. Financial literacy initiatives and tailored assistance for marginalised populations can enable individuals to make well-informed choices and safeguard their interests. Additional layers of protection can be implemented through the use of technological measures such as multi-factor authentication and scam detection software.
Methodology
This section presents a robust methodology with a view to ensuring that all the details of scams in the UK are well studied. The data analysis techniques that are at the forefront of technology and the ethical principles that are being upheld aim at overcoming the inherent research challenges and providing important information on scam identification and mitigation.The research utilises the methodological approach of mixed methods, allowing for an in-depth study of the issue. This method uses both quantitative and qualitative data from research articles and social media sites. The quantitative data comes from Action Fraud, the office for national statistics referrals to the National Fraud Intelligence Bureau (NFIB), Cifas, and UK Finance. The qualitative data comes from the same sources.
This research is unique in that it applies a predictive data model with a web-based application, which is used to calculate the possibility of an email being a scam. It is therefore a novel approach to email scam detection. The quantitative data is subjected to statistical analytical methods to detect any trends, relationships, and patterns that are present in the reported offences. This report provides a quantitative way of grasping the degree and nature of fraudulent activities in the UK. At the same time, qualitative data drawn from social and academic sources undergoes content and theme analysis to get a comprehensive picture of the damages caused to victims, geographical areas, public opinions about law enforcement, victim stories, and the tactics used in scams. By integrating the findings of these two analyses, the research becomes more scientific, and the contextual background is further deepened. Through the integration of quantitative and qualitative data, this research will be able to provide a comprehensive insight into scams in the UK. Through the calculation of the size of the issue, the digging out of the experiences of the victims, and the exploration of machine learning solutions to identify the trends and build predictions, this comprehensive strategy will give the right knowledge and the necessary tools to deal with these deceptive behaviours and protect vulnerable persons. By doing a comprehensive analysis like this, shed light on the complex issue of online fraud and equip people with the knowledge to confidently and safely traverse the digital landscape.
Developing a SPAM Email Detection System
In this application, machine learning classifiers for the identification of spam over non-spam (ham) emails are compared and evaluated in the realm of spam classification. The Python programming language and its associated data science libraries, especially Scikit-learn, which is famous for its vast range of tools for statistical modelling (Rosiene and Pe Rosiene, 2020), will be used in this study. The study's methodology begins with data preparation, which is the most important step in the machine learning process. Missing values are dealt with, and categorical variables are converted to numbers in order to make them available for the algorithm (Yang and Qiuxiang, 2022).
Comparative Analysis of Detection Models
This research investigates the performance of six machine learning models: logistic regression, naive bayes, K-nearest neighbours, decision tree classifiers, random forests, and SVMs (support vector machines). The performance of each model is meticulously assessed using various metrics: accuracy, precision, recall, and the F1 metric. Such metrics give information on how effective each classifier is at discriminating spam from ham emails. The accuracy is the ratio of truly predicted observations to the total observations. Accuracy is the proportion of correctly predicted positive observations to the overall predicted positive ones. Classification, also known as sensitivity, is the measure of correctly predicted positive observations over all observations in the actual class. The F1 score is the weighted mean of precision recall, which gives a single measure of the accuracy of the test(Sokolova and Lapalme, 2009).
1.Logistic Regression
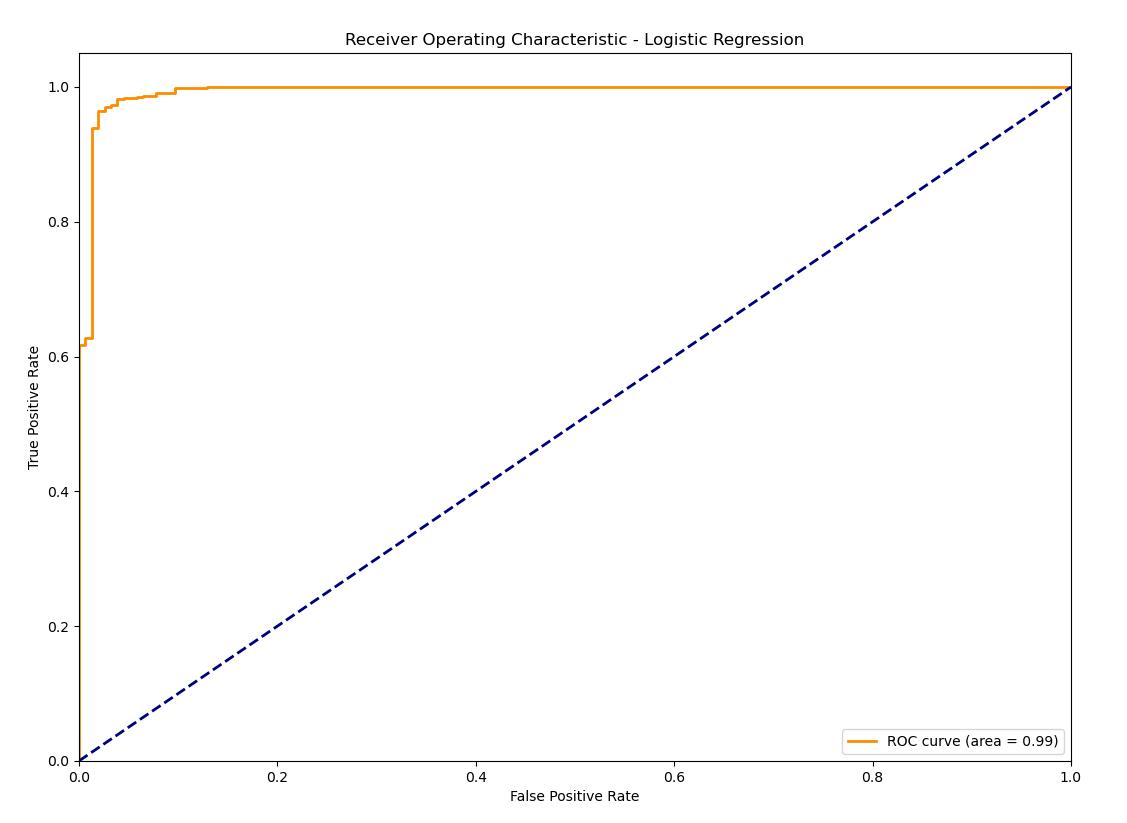
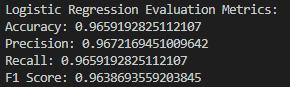
The ROC curve shows Logistic Regression a high area under the curve (AUC), revealing a good performance in terms of distinguishing spam from ham emails. The curve follows the upper left corner quite well, which means the true positive rate and false positive rate are in a balance. Logistic Regression is an excellent method, providing a high accuracy along with balanced metrics, and therefore, it can be safely used for binary classification tasks.
- Naive Bayes
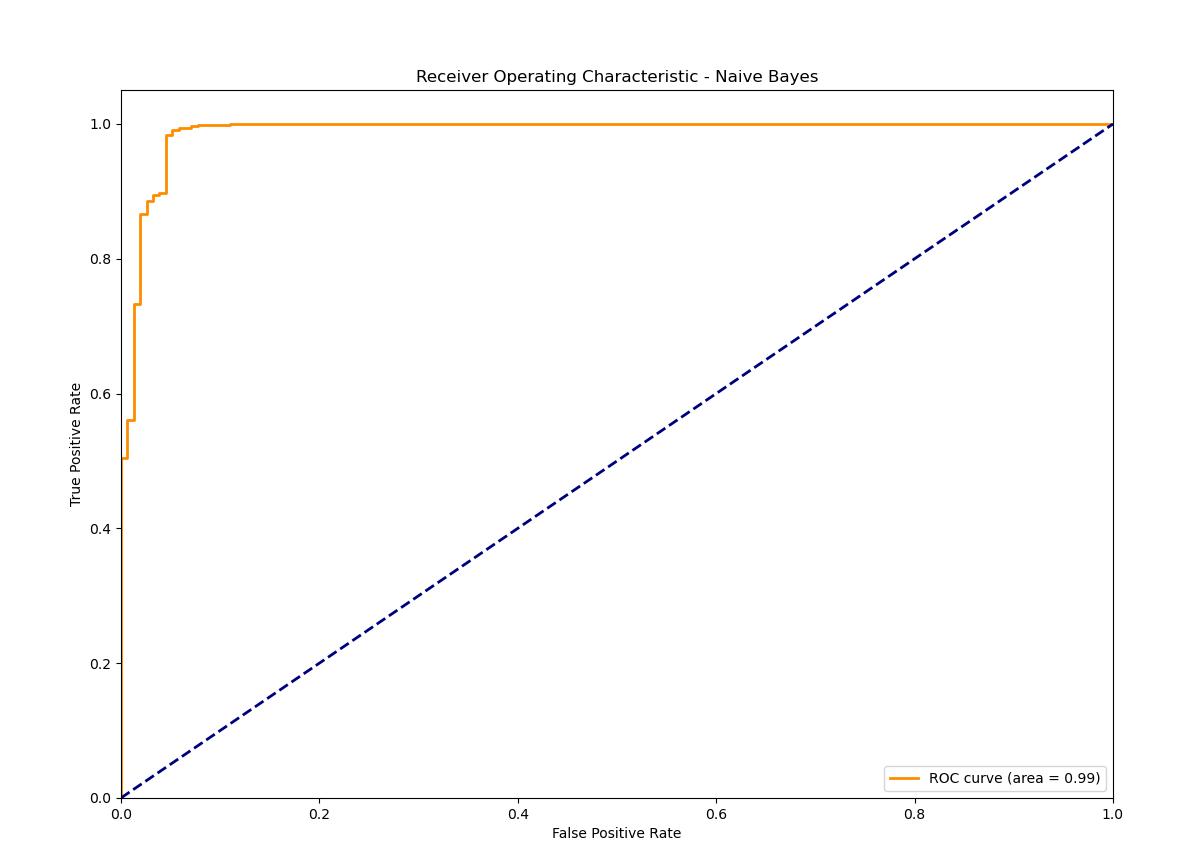
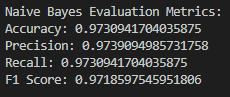
ROC Plot: The ROC curve of the Naive Bayes model actually gives a higher AUC than the Logistic Regression model, and so it is marginally better at differentiating spam and ham. The capability of applying text classification through this approach is largely attributable to that assumption of feature independence. Naive Bayes achieves the best results and outperforms Logistic Regression slightly in all metrics, which is evidence of its ability to classify texts correctly, especially due to it assuming the independency of features.
- K-Nearest Neighbors (KNN)
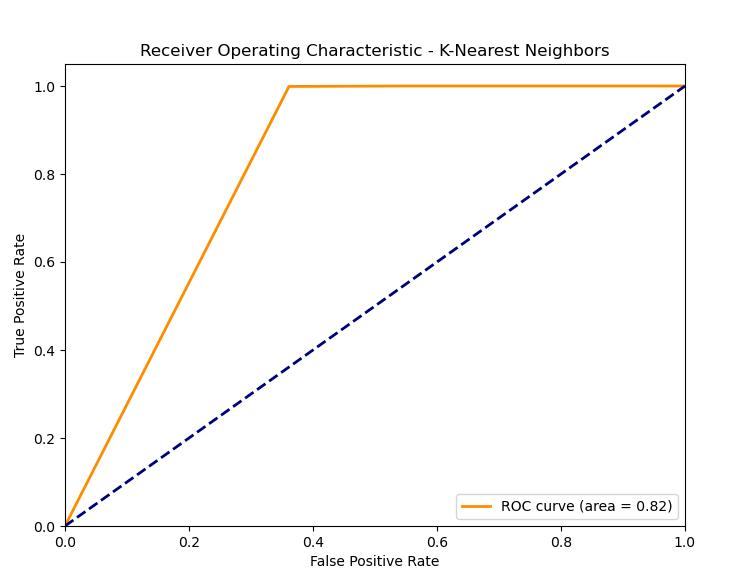
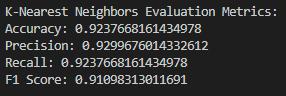
ROC Plot: The AUC of ROC curve for KNN is less than the others models, it can be inferred that KNN is not the best suited model for this task. There could be a possibility of this because KNN uses the proximity of the similar data points to reach the decision, which is not less difficult for the text data due to the high dimensional space. K-Nearest Neighbors has lower scores in comparison to other models. This might be the case, since it often uses the closeness of similar data points that is not a very efficient way when it comes to high dimensional text data.
- Decision Tree
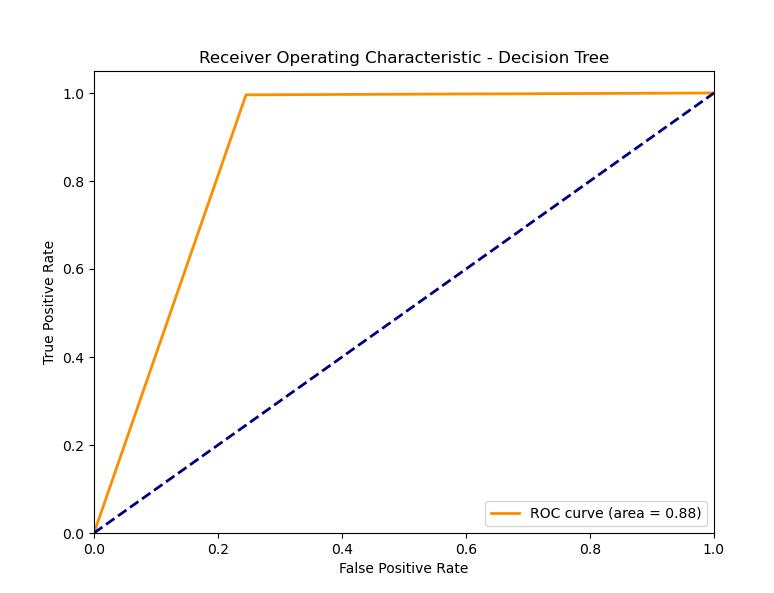
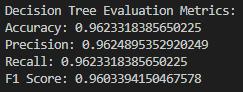
ROC Plot: It has a good ROC curve, with the AUC being not as good as some other models. This might indicate a satisfactory but not outstanding result: maybe a decision boundary could be too simple or the network overfits. The decision tree model is a robust model that is both accurate and generalizes well. Hence, this model somewhat slackens its performance in comparison with others, maybe because of overfitting or the simplicity of the decision boundaries.
- Random Forests
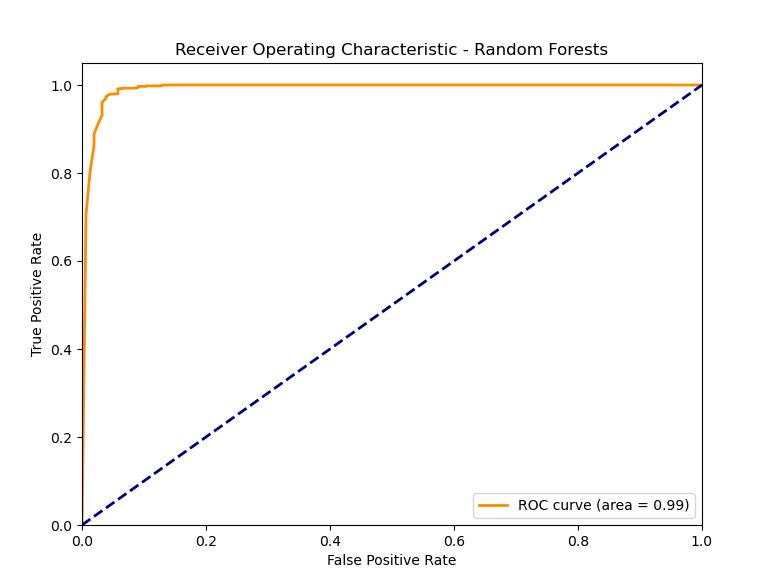
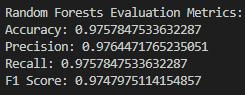
ROC Plot: The Random Forest model is characterized by the highest AUC score among the models under consideration, which implies high accuracy in classification. The superiority of this model is based on the ensemble approach, which consequently will provide more robustness and reduce the probability of an overfitting occurrence. The Random Forests surpass the Decision Tree model by means of an ensemble of trees, which substantially boost precision and immunity to overfitting.
- Support Vector Machines (SVM)
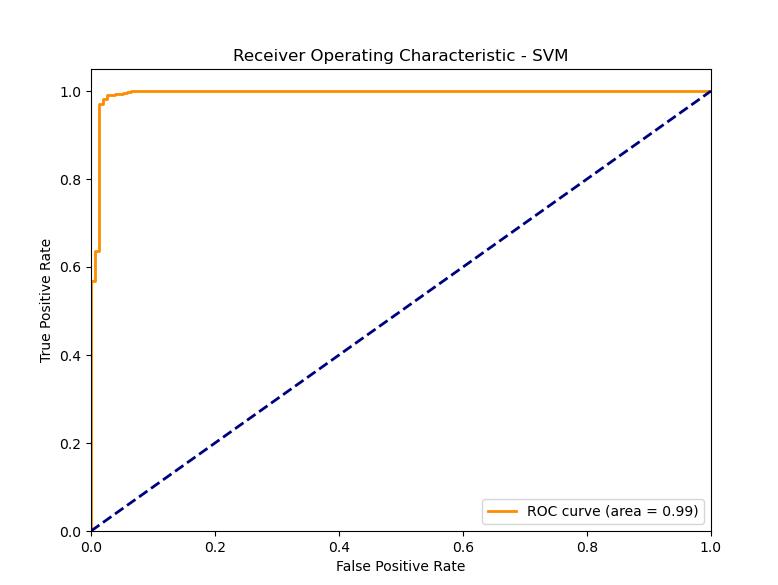
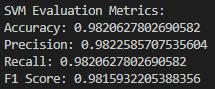
ROC Plot: The SVM model is the strongest in terms of the ROC curve, as the curve shows the highest AUC, indicating the best distinction among spam and ham emails. This unique capability can be attributed to its mechanism of coming up with the best hyperplane that maximally dissects the two classes. SVM reached the best results on all measures, proving it is able to generate the best hyperplanes for such problems through linear kernels.
Selection of Dataset
This research involved collecting data from multiple sources to thoroughly investigate the intricacies of scams and exhaustively document every aspect of these fraudulent actions, including their physical and emotional impact on victims. Since the beginning, this project has been conducted using original and correct data from authorized parties like Action Fraud, CIFAS national statistics, and the National Fraud Intelligence Bureau (NFIB), allowing this research to be more genuine and informative. Here are a few examples of the categories that were examined to determine the final results.
Data for this study is primarily obtained from the Office for National Statistics (ONS), which is the preeminent independent producer of official statistics in the United Kingdom and is officially recognised as its national statistical institute. The publication and distribution of data concerning the economic, social, and demographic dimensions of the nation across different territorial levels falls within the jurisdiction of the ONS. Furthermore, the administration of the decennial census for England and Wales by the ONS constitutes a substantial contribution to the existing corpus of demographic data.
The ONS, with the aim of augmenting the transparency and accountability of governmental operations, is dedicated to expanding the accessibility of information pertaining to its organizational framework. Information regarding fiscal expenditures and organizational hierarchy is disclosed publicly for this purpose. Additionally, the Freedom of Information Act grants the general public the right to request access to supplementary data.
To guarantee the production of high-quality statistics, the ONS employs a diverse range of methodologies. The documentation of these methodologies is comprehensive in reports on quality and methodology information.(Quality and methodology information National Statistics,) The purpose of these reports is to provide users with an understanding of the data's merits and drawbacks in accordance with the five Eurostat quality dimensions: coherence and comparability, relevance, accuracy, timeliness and punctuality, accessibility and clarity. The purpose of such comprehensive reporting is to furnish stakeholders with the essential framework required to assess the suitability of the data for particular applications. Subject to the stipulations of the Open Government Licence and the UK Government Licencing Framework, which were established on September 30, 2010, the ONS material may be used and reused without restriction for commercial or personal purposes, in accordance with the terms and conditions of data usage (Licences - Office for National Statistics).
Approaches to Analysis each dataset
fraud and computer misuse in the UK
To analysis all the data in the Frad and computer misuse data set Used the dataset of the National Statistics Office and Action Fraud.(Office for National Statistics, 2023) (Action Fraud,2023)The main analytical instrument employed in this venture is panda’s library, which offers robust data manipulation and analysis capabilities(Mckinney, 2010). The analysis begins with the import of the dataset from an excel file on the user's local drive into a pandas Data Frame. Here is the first step that is the basis for the data cleaning and editing. The data cleaning phase is made up of removing the columns and rows that have redundant percentage change information or null values. The attention is sharply focused on the particular groups of offences in the study that are of interest—Bank and Credit Account Fraud, Consumer and Retail Fraud, Advance Fee Fraud, and Other Fraud. As a result, dataset that is more targeted and refined. The procedure is not done once but over and over again on many tables within the database; it mirrors the complex terrain of computer misuse as well as the usual crimes such as fraud. The data from these varied offence types are subsequently combined into a single Data Frame, thereby giving a comprehensive overview of the offence incidents occurring across various timeframes.
BY using the data set of the scams, the time series line plots generated by matplotlib, a plotting library, serve to visually depict the temporal dynamics of these offences. The visualisation effectively illustrates the patterns and trends present in the dataset, converting the unprocessed data into a comprehensible storyline that develops gradually (Hunter, 2007). Beyond a simple description, the study goes further and gets into the area of predicting with the statmodels package's SARIMAX model. This model is capable of reflecting both the patterns of time series data, as well as seasonality, which makes it a strong instrument for predicting the future on the basis of the historical statistics (Seabold and Perktold, 2010). The method of forecasting is applied to each crime group, providing a data-based estimate of crime numbers for the future. This exercise facilitates the connection between past trends and possible future situations, allowing policymakers to predict and equip themselves for the progression of fraudulent activities.
Personal and household characteristics of who were victims of fraud
Analysis Personal and household characteristics data from the Crime Survey for England and Wales (CSEW)(Office for National Statistics,2023), and the data from the Action Fraud which covers the fiscal year ending March 2023 and also used the first step is to import the panda’s library, which is a Python package that provides flexible data structures for working efficiently with structured data (Mckinney, 2010). Data from Excel files for different years (2022 and 2023) is loaded into separate Data Frames. Skipping the initial rows that do not contain relevant data is an important part of this process, as it ensures that the proper headers accurately reflect the dataset.
A curated list of desired characteristics is provided, which includes age groups, gender, ethnic backgrounds, employment status, and more. These characteristics are critical for understanding the various aspects of fraud victimisation. The Data Frames are then filtered to include only the desired characteristics, demonstrating a more focused and tailored approach to data analysis. The subsequent analytical step merges the 2019 and 2020 data based on the 'Personal characteristic,' allowing for a comparison of changes over time. A new Data Frame is then created, encapsulating the necessary columns and displaying the calculated changes, resulting in a clear year-on-year analysis of the demographic data regarding fraud victimisation. The statistical interpretation is refined further by using the Random Forest Regressor from the” sklearn.ensemble” module to build a predictive model within a pipeline that includes preprocessing steps such as imputation for missing values and one-hot encoding for categorical variables. The model is trained, and predictions are generated, providing insight into potential trends and patterns.
Linear Regression from the “sklearn.linear” is then used to forecast values based on the imputed and split data, enriching the analysis with predictive capabilities(Pedregosa FABIANPEDREGOSA et al., 2011). Visualisations are created with ‘’matplotlib.pyplot’’, which brings the data to life by providing a graphical representation of the comparison years as well as the predicted data for 2024. The visuals provide a more intuitive understanding of data trends and are especially useful for communicating complex information in an understandable format. The investigation expands to regional and area-specific analyses, shedding light on the geographic variations of fraud victimisation. The regional data is filtered and visualised, creating a compelling narrative about how the proportion of victims varies across different areas, whether urban or rural, or according to Output Area Classification.
Security measures taken to prevent fraud
In conducting a security measure against fraud in the UK, datasets from the Crime and Security Measures Appendix of the year 2023 were minutely collected from a wide range of individuals with different demographics. (National Fraud Initiative December, 2022) This approach enabled to carry out a longitudinal study where over the period of several years, from April 2017 to March 2023, could see a development of the landscape of fraud prevention strategies. Strict data processing, which involves cleaning and transformation to numbers, was performed to ensure that the conclusions were valid (Pedregosa FABIANPEDREGOSA et al., 2011). Analysis highlighted significant trends in the adoption rates of security measures, employing advanced statistical tools to quantitatively measure yearly changes in these practices. This data analysis revealed the fact that the methods of individuals and households to prevent fraud are changing due to the fact that the society is moving forward and the technologies develop and improve (Doe et al., 2019).
Research findings highlight the evolutionary the dynamic nature of security measures against fraud, with a clear trajectory towards more sophisticated and widespread adoption of preventive strategies over time. This reflects the increasing awareness and proactive stance of the public in combating fraud, a testament to the efficacy of ongoing educational and policy initiatives aimed at bolstering national security measures (Pedregosa FABIANPEDREGOSA et al., 2011). The study does not only track progress in the struggle against fraud, but also proves to be a valuable tool for lawmakers and security specialists for further developing more efficient fraud prevention strategies. This contribution, through the use of evidence-based statistics, establishes a safer and more secure society that is more capable of responding to the ever-evolving threats of fraud (Kaur, Gabrijelčič and Klobučar, 2023).
Emotional impact
The present work was focused on the emotional impact of fraud and computer misuse in the UK, and the data was gathered from the Crime Survey for England and Wales (CSEW) for the period of March 2017 to March 2023. The panda’s library was used to investigate and analyse each type of fraud and the emotional impact on the victims. The report summarized the data from various fraud categories such as bank and credit account fraud, consumer and retail fraud, and cyber fraud, as well as many other types of fraud.
The methodology used was to import data from specific sheets within an Excel file where each sheet represented the various aspects of emotional impact of frauds (e.g., Table_1a to Table_1j). The panda’s library was used for data cleaning, merging together the Data Frame, and analysis to determine the pattern of emotional impact over time. The procedure contained the section of emotional consequences (for example, "Very much," "Quite a lot," "Just a little," "Not affected") and more specific answers like anxiety, depression, and fear.
The study uncovered that the emotional impact of scamming goes beyond the financial loss and points out the importance of the creation of effective measures to fight against such cases and provide support for the victims. The study increases the level of knowledge about the psychological consequences of cybercrime, which points to the complicated emotional topography of modern society. This summary is an outline of the research methodology and main findings that utilizes the word limit to highlight the significance of data analysis in comprehending the emotional response towards fraud. Python panda’s library is a good example of how pandas can be used for data processing and analysis which makes it possible to handle large data sets and to derive meaningful conclusions.
Reason for contact with offender
A CSEW 2023 data analysis using Panda’s library of Python was conducted in order to examine the aspects that lead to contact with offenders in the UK. The process considered the importing of data from specified sheets and cleaning it by replacing the "[x]" with 0 and aggregating it to obtain the patterns and trends relating to the fraud and computer misuse incidents (McKinney, 2018). The resulting dataset provided with a great foundation for further analysis and visualization methods, which consequently allowed to see the evolving pattern of these offenses over time.
Data consolidation enabled the creation of insightful graphics utilizing Matplotlib which showed the aggregated consequences of these crimes from 2017 to 2023(Hunter, 2007) . Used advanced statistics methods, such as Random Forest Regression, to model and predict future offences by analysing historical data trends (Pedregosa et al., 2012). This shows a proactive approach to understanding and mitigating such crimes. This analytical journey clearly brings home the point that data science plays a crucial role in unveiling the complexities behind inmate contacts, and the predictive analytics in turn serve to formulate effective preventive strategies. It's a proof of the power of data analysis in this regard, which can bring to light the ways society gets safer, thus introducing the idea of technology, criminology, and policy-making.
Financial loss suffered by victims of fraud
This analysis on the financial losses experienced by the victims of fraud in the UK uses the Python libraries pandas for data manipulation, matplotlib, and seaborn for data visualization to make it clear how many people were affected by the crime and what the consequences of the fraud were. The dataset” fraudandcomputermisuse23.xlsx” is a detailed record of events from 2016 to 2023 which has been divided into the sheets, each showing a different aspect of the fraud incidents and financial losses. The method of the analysis is highlighted by the construction of heatmaps, which allows representing the distribution and the magnitude of the losses during the years, giving a total view of the financial consequences.
8 Number of frauds by police force area
The analysis of scam cases throughout UK police areas is a domain, where the libraries of Python, such as pandas for data treatment and seaborn for visualization, play a very important part. By careful data cleansing that includes the removal of unnecessary columns and standardization of area names, and then classification of the areas for the purpose of consistent analysis, a stage is set for thorough analysis. A diagrammatic exhibition created by seaborn displays the variation in scam events in different regions and time frames which gives a clear insight of the fraud in the UK. This approach sheds light on the role of structured data analysis in revealing patterns and providing strategies for scam eradication.
Research Findings and Analysis
Evaluating the SPAM Email Detection System
In the context of a spam email detection application, the process involves several methodical steps using machine learning algorithms, specifically Support Vector Machines (SVM) with a linear kernel. This was built with the different models to evaluate the accuracy, and the Support Victor machine was the best model when compare the accuracy because of that SVM was the selected model to build this application.
These steps are as follows:
- Data Acquisition: Gathering and labelling a dataset that includes both spam and non-spam emails, crucial for training and evaluating the machine learning model.
- Data Preprocessing: Cleaning and transforming the text data to enable the learning algorithm to process the information effectively. This includes normalization, tokenization, and feature extraction.
- Model Selection: Choosing an appropriate machine learning model to classify emails. Support Vector Machines (SVM) are chosen for their effectiveness in high-dimensional spaces and their ability to model non-linear decision boundaries using different kernels.
- Model Training: Utilizing the “sklearn” library's SVC class with a linear kernel to train the SVM model on the pre-processed training data. Training involves adjusting the model parameters to minimize prediction errors.
- Prediction: Finally, the trained SVM model is applied to make the decisions on the class (spam or non-spam) of new email entries. Predictive accuracy has been recognized as the main performance evaluation indicator.
- Evaluation: The assessment metrics of accuracy, precision, recall, and the Receiver Operating Characteristic (ROC) curve can be used in such a way. The ROC curve is a plot that represents the true positive rate versus the false positive rate at various cut-off values, and area under curve (AUC) serves as an index of the model's ability to distinguish between the classes.
- Performance Visualization: Tracing the ROC curve using matplotlib, highlighting the model's performance in spam detection. A model which has an AUC close to 1.0 is a good performer.
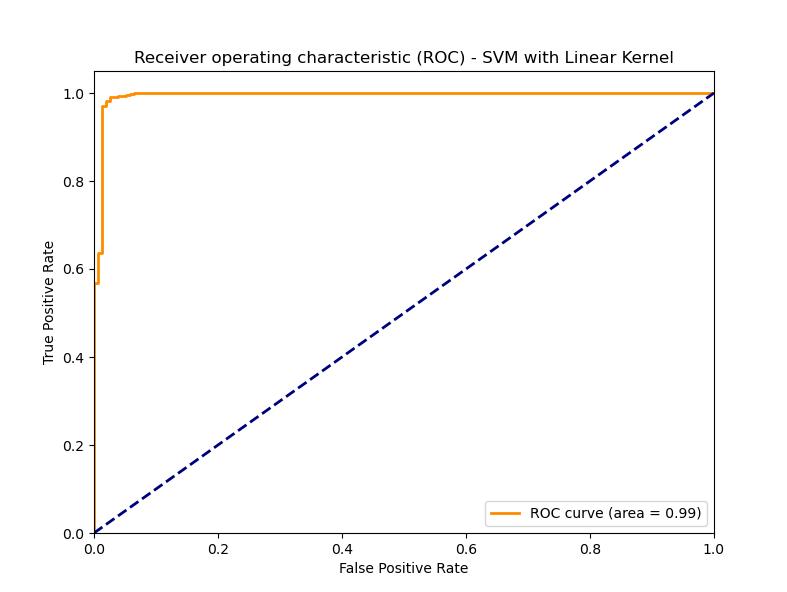
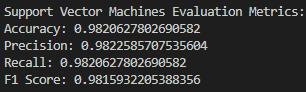
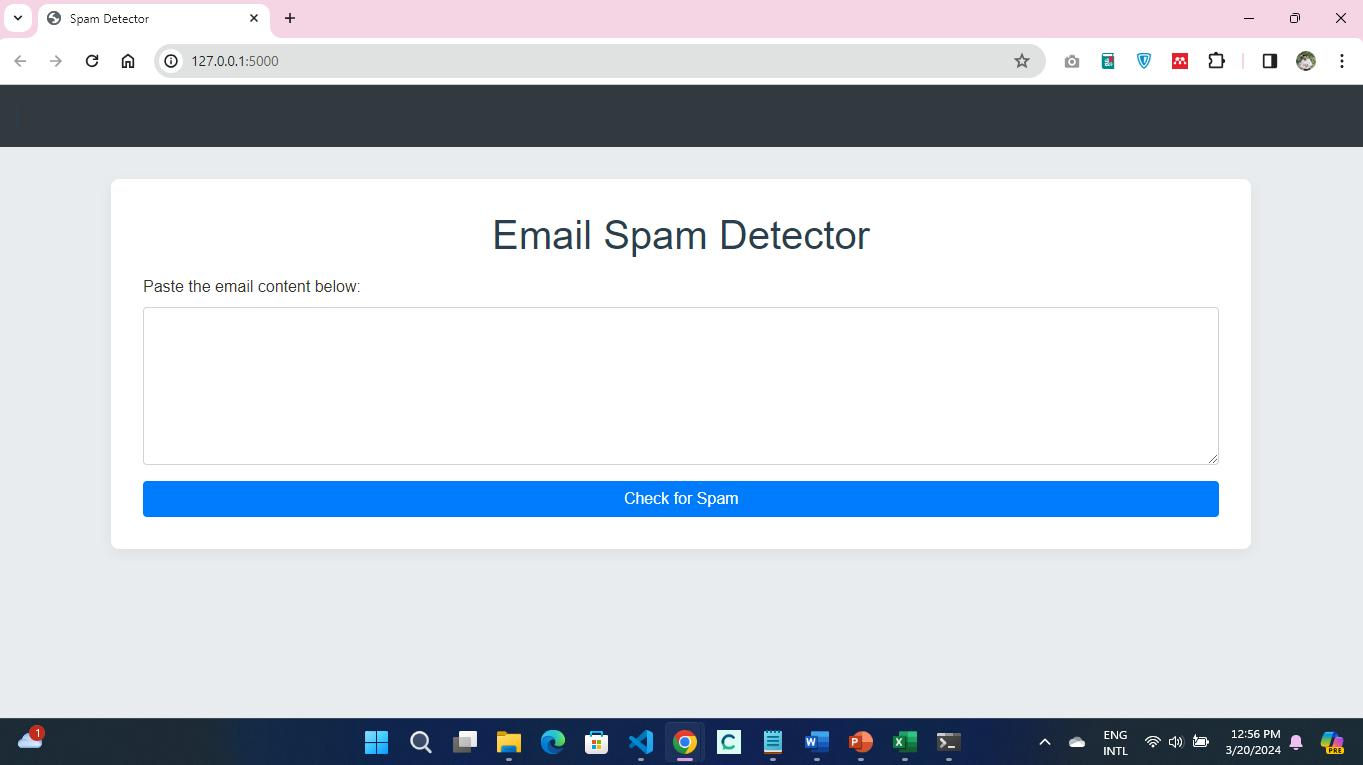
The spam detection application will provide users with a box where they will be required to enter the contents of an email, which will then be processed through an SVM classification model. The web application is the interface used to display the classification results, which are either classified as spam or legitimate. The application operates on a local server, as denoted by the IP address 127.0.0.1:5000, which indicates that the application was developed on the local server for development purposes. In this regard, it uses a web framework, presumably Flask, for its backend operations, which is the popular option for such tasks because of its lightweight and flexible nature.
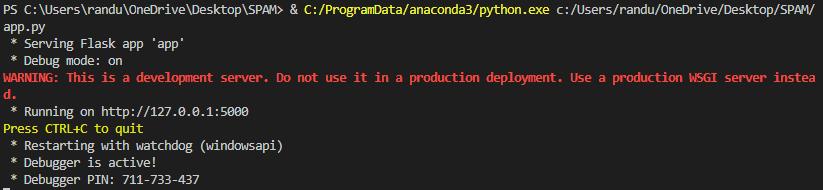
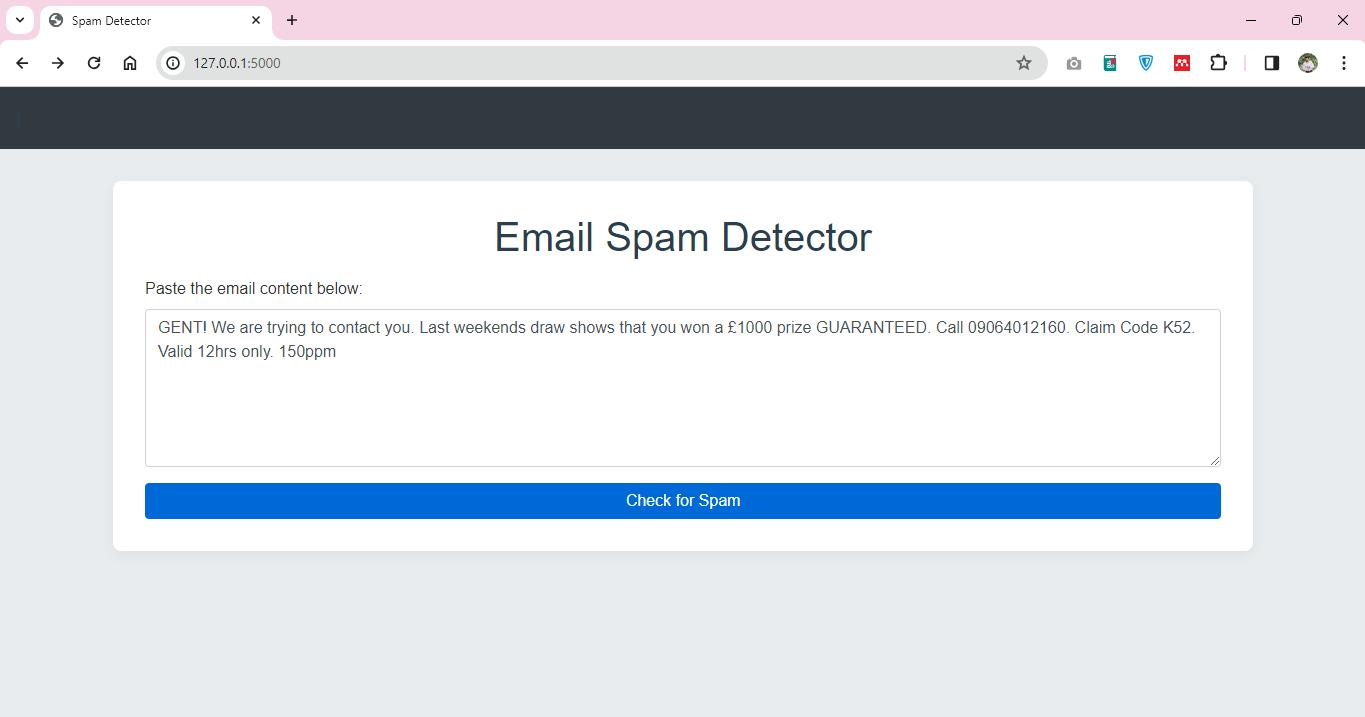
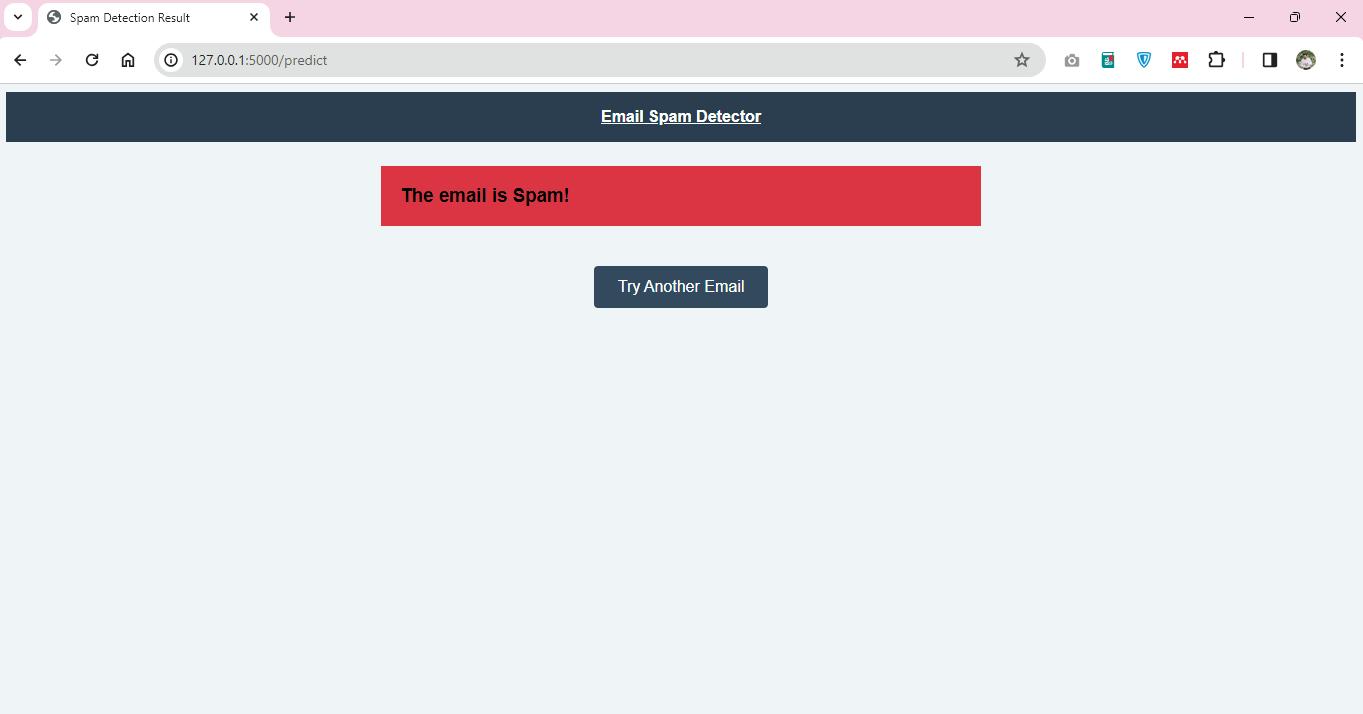
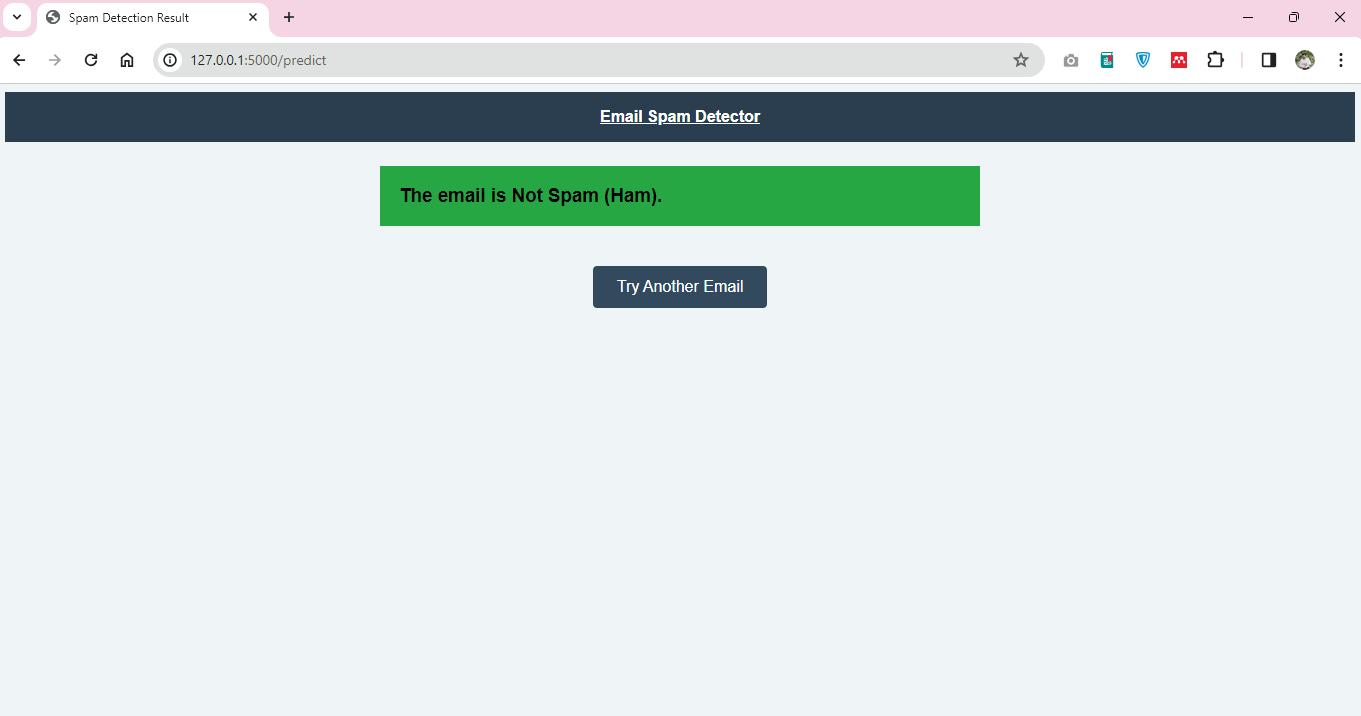
When executing the spam detection application, the following output is generated in the console, indicating that the Flask app named 'app' is in active development mode and running on the local server. Debug mode in Flask enables the coders to see the changes to the code in real-time while the server is running and offers an in-browser debugger for problem-solving.
The code snippet and the plot of ROC curve show that the SVM model has a high discriminative ability, which is manifested in the AUC of 0.99, that means it is a good classifier of spam emails. A low false positive rate. The interface of the web application simplifies the process for users, as they can perform the classification of emails in a short time. The spam detection algorithm has to be complex to be able to recognize the changing spam techniques. SVMs are one of the machine learning models that belong to a arms race between spammers and the spam filtering service providers, requiring constant training and updating for better accuracy.
In summary, the ROC curve is a measure of the accuracy of a support vector machine model in classifying emails, and the web app interface is an easy-to-use tool for the technology. The next research agenda is to improve such models if they will be able to keep pace with the dynamism of spam emails.
Evolution of Fraudulent Offences and Computer Misuse with Predictions
Fraud and computer misuse in the UK
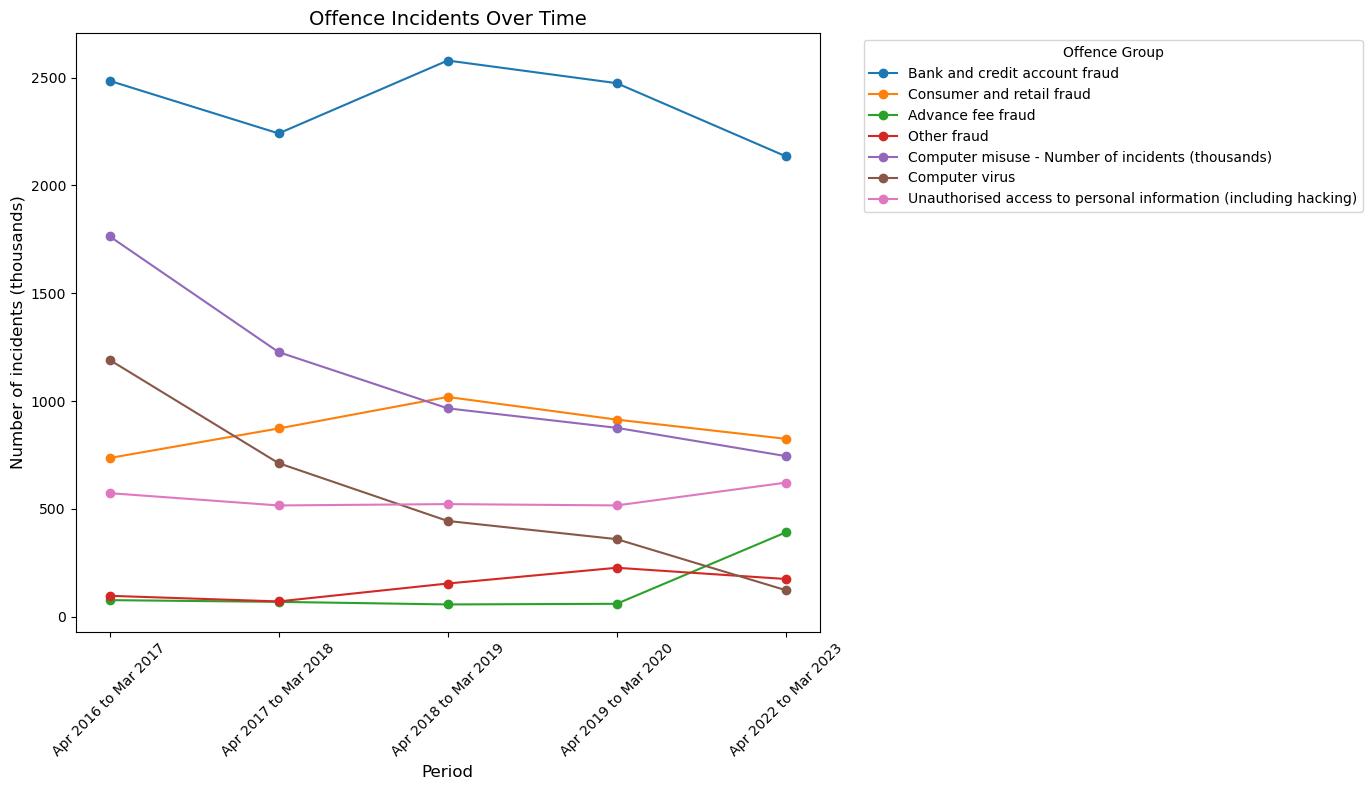
The line chart shows the summarize number of reported fraud and computer misuse offences in the UK for the period 2016-2023, from April 2016 to March 2023. A significant trend is the reduction in bank and credit fraud cases from 2,485 (thousands) in 2016-2017 to 2,135 in 2022-2023. Besides, there is a tremendous increase in the number of victims of advance fee fraud from 77 to 391 within the same time frame.
Consumer and retail fraud incidences are not on constant rise but rather show a slight drop from 736 to 825. The other variety of fraud also shows a little increase from 97 to 175 incidents. In the case of computer misuse, can see a significant decline from 1,764 incidents to 745, which may point to decrease in computer virus incidents from 1,191 to 123, suggesting improved cybersecurity measures. But, the leakage of personal data increases from 573 to 622 incidents which indicates the growing problems in ensuring security of personal data. These patterns show that cyber threats are changing, and that to fight them, strategies for fraud prevention and cybersecurity defences should be adapted accordingly. The statistics reflect a continuous battle against cybercrime, and hence, emphasize the need for the companies to be very vigilant and always update their security protocols.
Predictions
ARIMA model is the best model in the time-series analysis because it can be used for forecasting data with trends, seasonality and other complex features. Dong et al. (2017) stress the ARIMA model's great strength in the short-term forecasting capability, thus it is the first priority and competitive method to predict the stock market investment decisions(Dong, Li and Gong, 2017) and also Liu et al. (2016) highlight the flexible and statistically properties of ARIMA to be the It can include both autoregressive and moving average components that ARIMA can then use to model most of the time series data types accurately.(Liu et al., 2016)
On the contrary, models such as logistic regression are not as well-suited for time series data as they don't capture the time-dependent structure of time series data as their focus is on the relationship between a dependent variable and one or more independent variables exclusively. Ranganathan et al. (2017) highlighted the most of the misuses made by people while doing the statistical analysis. They highlighted the fact that the methods like this are not very good at dealing with time-independent structures and they argue the necessity for better models, which will be able to properly capture these changes(Ranganathan, Pramesh and Aggarwal, 2017). In addition, Abbott (1985) showed that logistic regression was not adequate for survival analysis, a field which is significant for time series data, which led to the further highlighting of the method's inadequacies for event time data.(Abbott, 1985). ARIMA is superior to the other models because of time-series patterns and trends that are needed for the accurate predictions which are of great importance for the forecasting tasks. This is a direct consequence of the logistic regression's inability to predict outcomes of a binary nature and to make allowance for the sequential nature and autocorrelation of time series data.
To follow below are the forecast for the "April 2023 to March 2024" period based on the past data. This code uses ARIMA, a forecasting method that uses time series to predict future offence rates. The machine learning model is built by going through historical data on different offences until the year 2016, and then it sets yearly trends. Parameters (1, 1, 1) usually let the model to look at the past, the trends, as well as the error influence. In April 2023 to March 2024, the foretastes are different on some fraud and computer hacking cases, showing one category of decrease. Through the small model, ARIMA has shown its effectiveness in getting the crime patterns and therefore taking the proactive measures against the future offences.
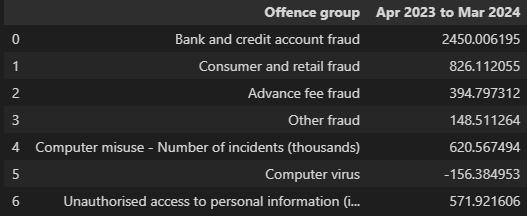
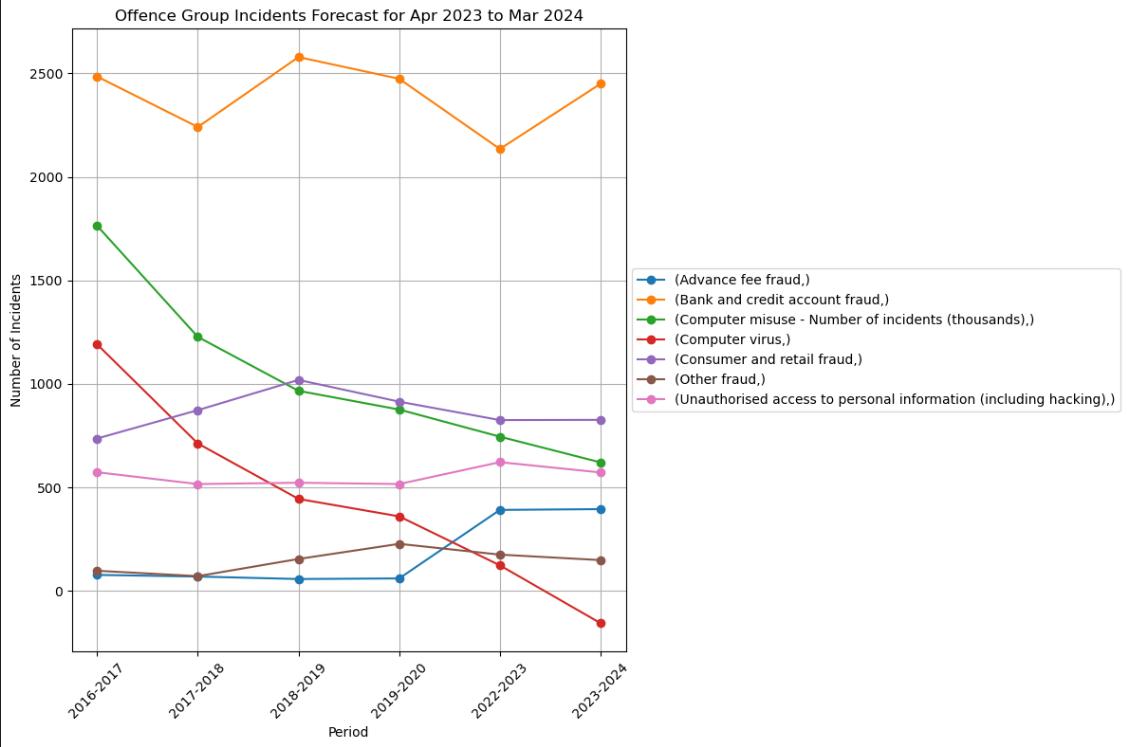
The graph and dataset presented offer a comprehensive projection of diverse computer misuse and fraud offences in the United Kingdom spanning the time frame of April 2023 to March 2024. Based on an analysis of moving averages, autoregression, and differencing, ARIMA modelling predicts that credit and bank card fraud will be the most prevalent, accounting for 2,450.01 incidents in total. This finding emphasises the critical need for enhanced security measures in the financial sector. According to a significant estimate by (Bainbridge, 2007) consumer and retail fraud is the second most prevalent type of fraudulent activity, involving 826.11 cases per year. This number poses considerable dangers for both businesses and consumers. Predicted to account for 394.80 and 148.51 incidents, respectively, advance fee fraud and other types of fraud indicate that these domains continue to face formidable obstacles (Murray, 2019). Unexpectedly, the data suggests a predicted decrease in occurrences of computer viruses, which may indicate a genuine decline or a data anomaly necessitating additional scrutiny. With 571.92 incidents of unauthorised access to personal information anticipated, the report concludes by highlighting ongoing concerns regarding data protection (Correia, 2019). The aforementioned predictive analysis functions as a critical metric for law enforcement and cybersecurity experts in order to formulate strategic plans and distribute resources in an effort to thwart cybercrime and fraud. In order to attain a thorough comprehension that surpasses the scope of this analysis, scholarly research may provide insights grounded in empirical evidence.
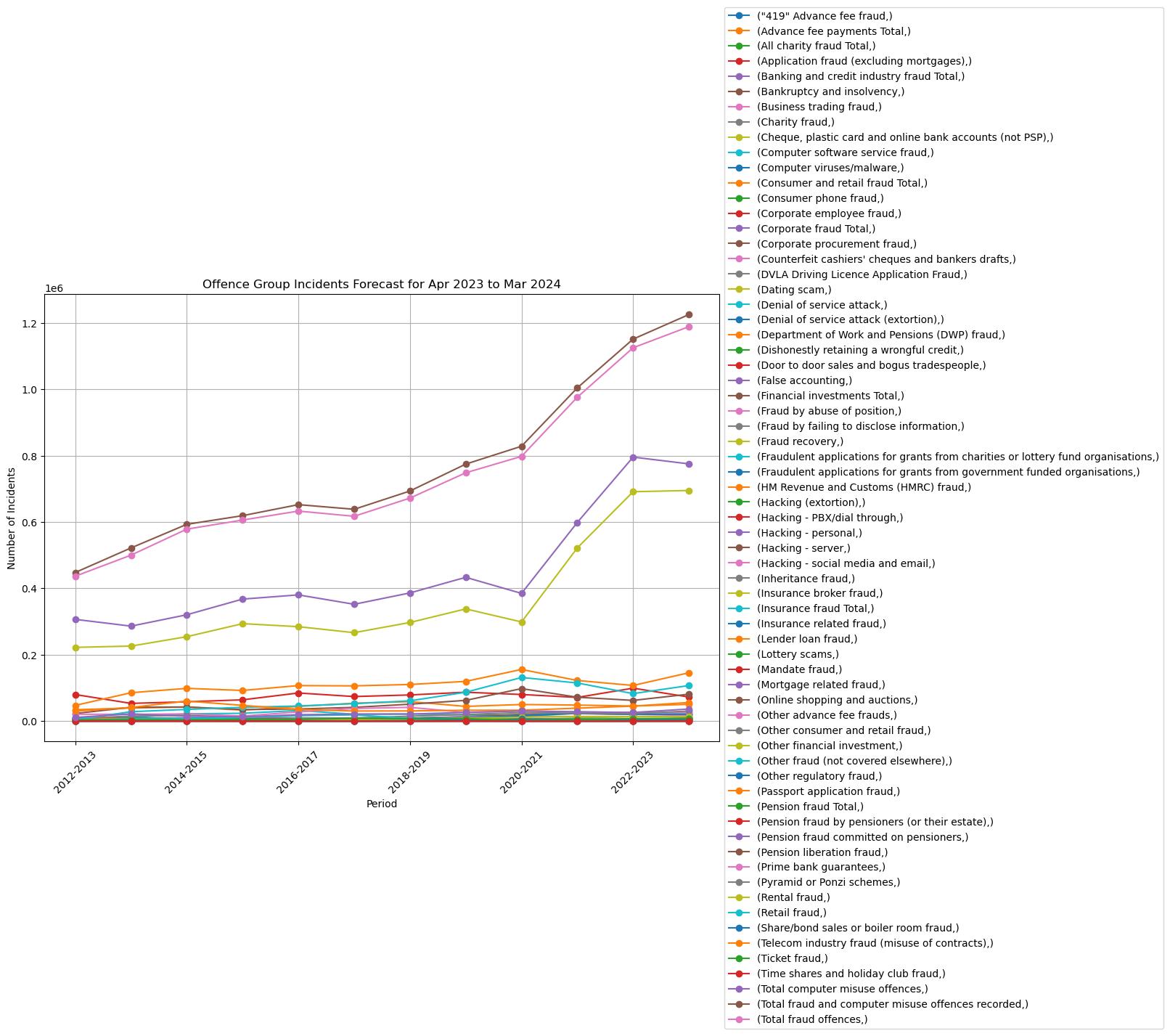
The line graph is the uncategorized data set with all the categorised projects and the anticipated trends in various fraud and computer misuse incidents in the UK from April 2023 to March 2024. The data visualisation demonstrates a varied forecast for different types of offences. This graph is critical for understanding and preparing for emerging threats in cybersecurity and fraud prevention.
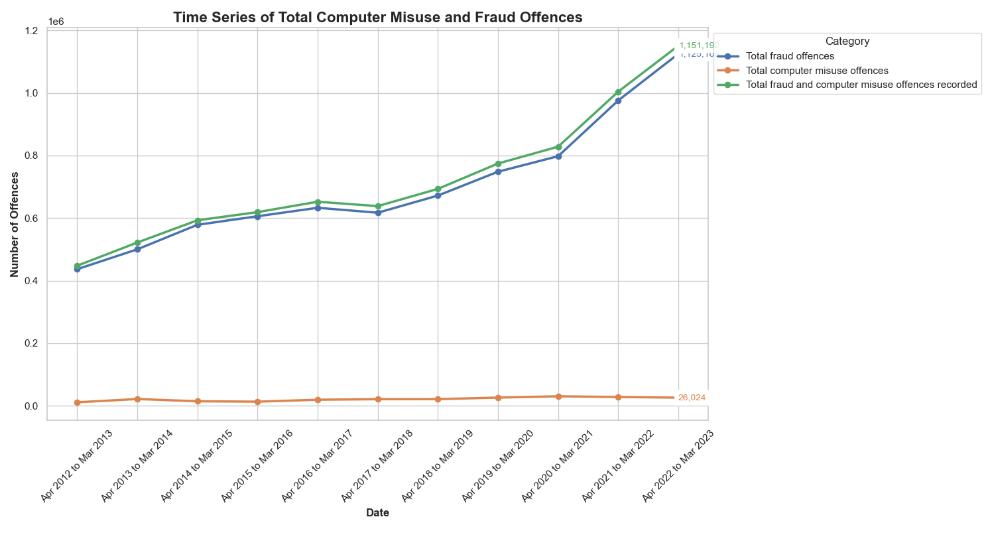
From April 2012 to March 2023, the line graph illustrates a consistent upward trend in the aggregate number of computer misuse and fraud offences committed in the United Kingdom. Although there has been a notable and sustained increase in the overall count of fraudulent offences, the growth rate of computer misuse offences has been comparatively moderate, culminating in a substantial peak in the combined total offences during the final period.
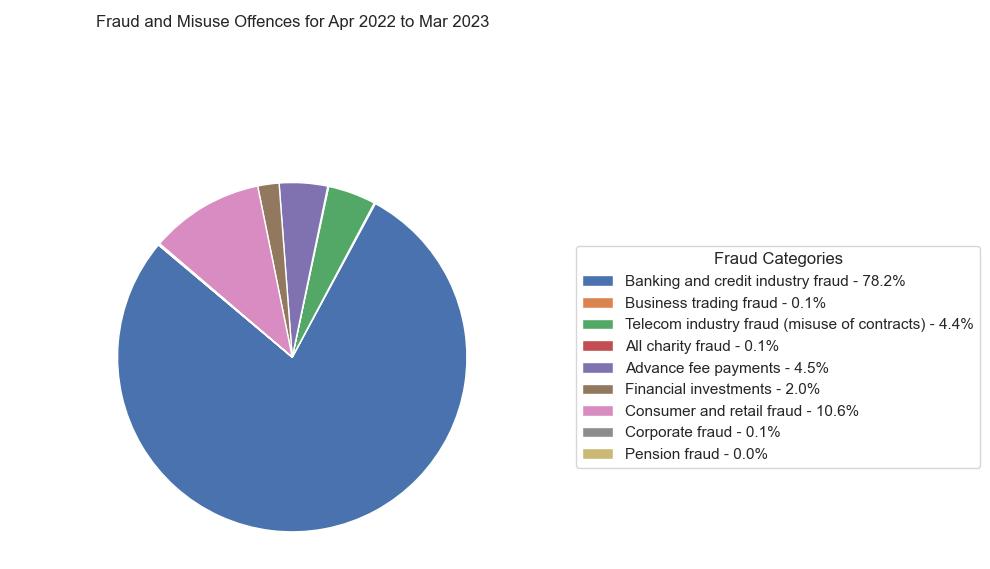
the pie chart illustrates the distribution of various fraud and computer misuse offences reported from April 2022 to March 2023 in the UK, with banking and credit industry fraud being the most prevalent at 78.2%. Consumer and retail fraud follows at 10.6%, while other categories such as telecom industry fraud, advance fee payments, and financial investments fraud contribute smaller percentages to the total.
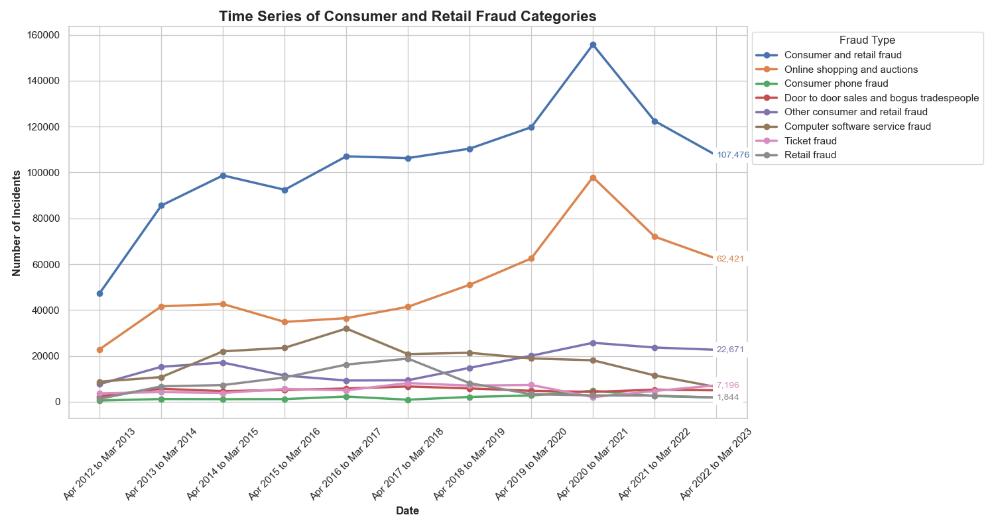
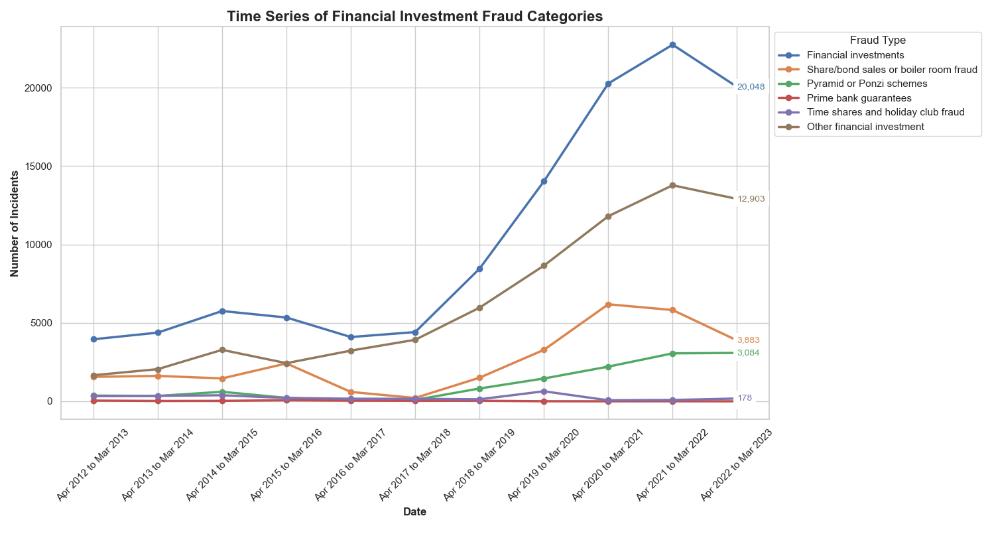
An Insight into the Personal and household characteristics of who were victims of fraud
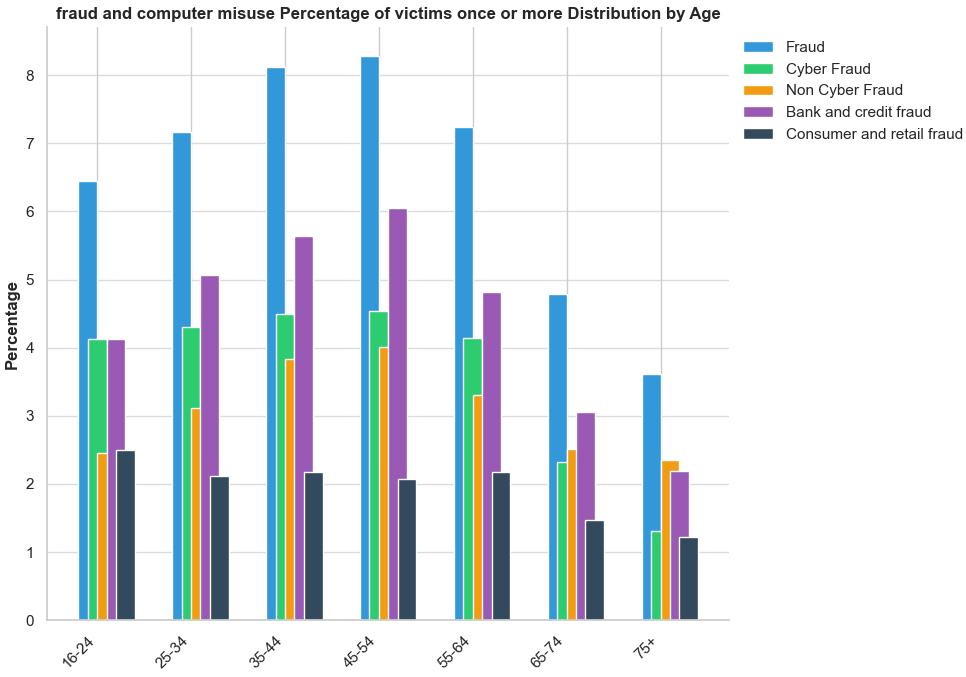
The bar chart illustrates the proportion of victims of fraud and computer misuse according to age group. It is evident that younger individuals (16–44) are more susceptible to fraudulent activities, particularly cyber fraud, in comparison to older age groups (65+). In contrast to the 35-44 age bracket, which experiences the highest incidence of both overall and cyber fraud, the 45-54 age bracket is where bank and credit fraud, as well as consumer and retail fraud, reach their peak.
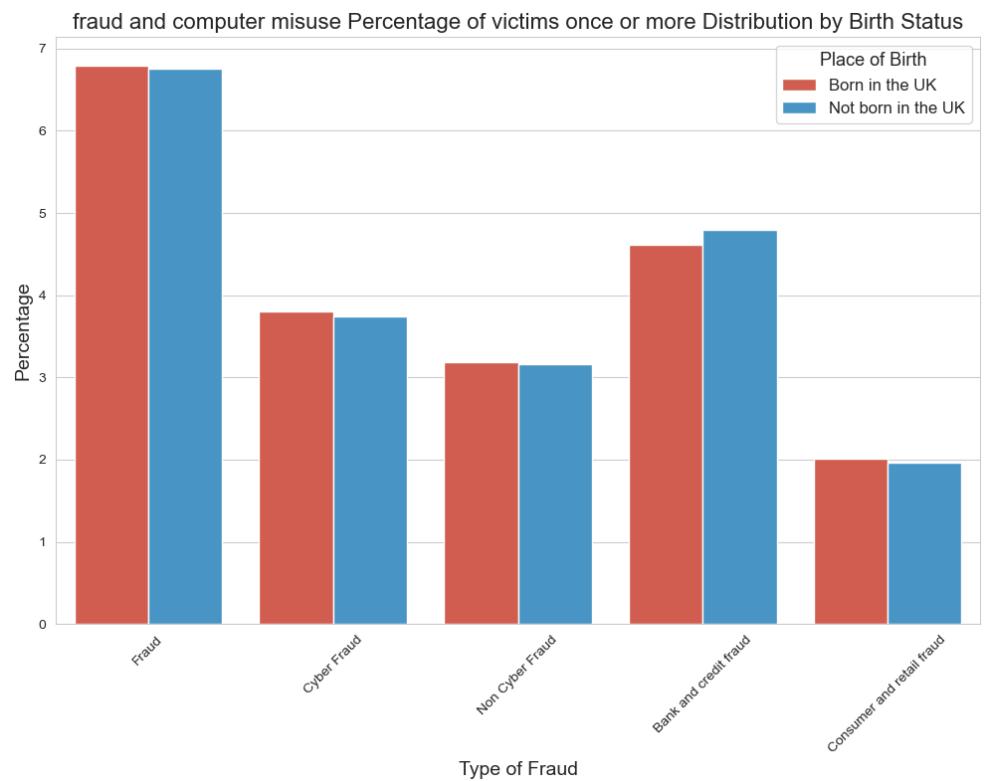
The bar chart presents the proportion of individuals whose place of birth corresponds to the number of instances in which they have fallen prey to fraud or computer misuse in the United Kingdom. There appears to be a marginal increase in the susceptibility of individuals born in the UK to fraud compared to those not born in the UK, with cyber fraud and bank and credit fraud being particularly prevalent.
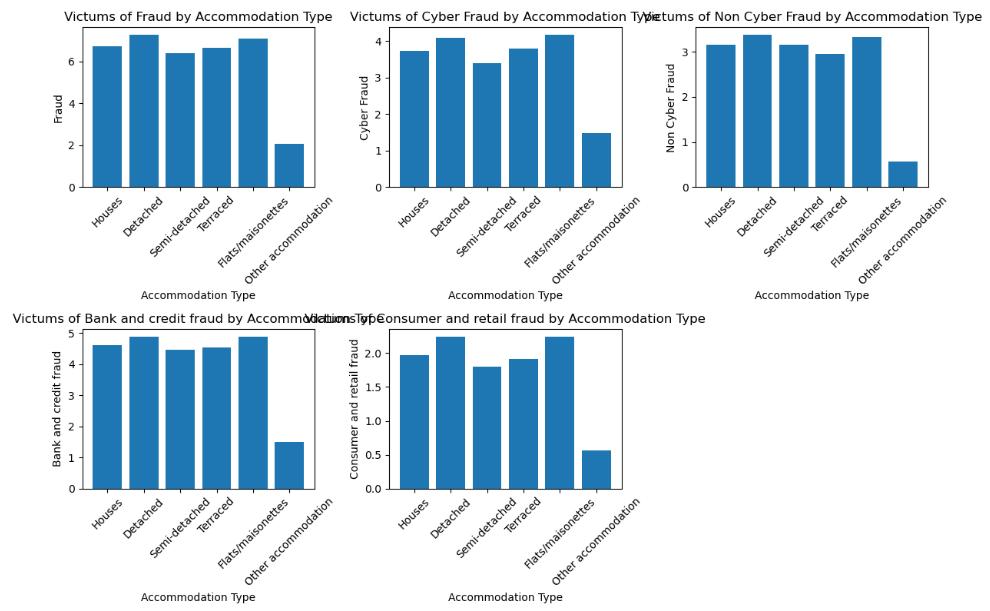
General Fraud: Houses are the most frequently targeted property for general fraud, with detached accommodations being a close second. There are fewer reports of incidents from semi-detached and terraced accommodations, while apartments and other types of lodgings experience the least impact.
Cyber Fraud: Detached accommodations are the most susceptible to cyber fraud, followed closely by houses. The inclination gradually declines in favour of alternative lodging options, with apartments and other forms of accommodations receiving the least attention.
Non-Cyber Fraud: Similar to cyber fraud, the pattern for non-cyber fraud demonstrates a discernible decline in flats and other accommodations, while detached houses and detached dwellings remain the most susceptible.
Bank and Credit Fraud: exhibits a higher incidence rate in residential properties, while its occurrences decline substantially in detached accommodations and remain relatively uniform among the remaining types of accommodations.
Consumer and Retail Fraud: most prevalent in residential properties, with detached accommodations reporting the second highest number of incidents. Condominiums and similar lodgings are least impacted.
Overall, the consistent theme across all charts is that houses, followed by detached accommodations, are the most common targets for various types of fraud. The data suggests a potential correlation between accommodation type and fraud risk, with ‘houses’ being a common high-risk category across all forms of fraud depicted.
Prevention and Security Measures
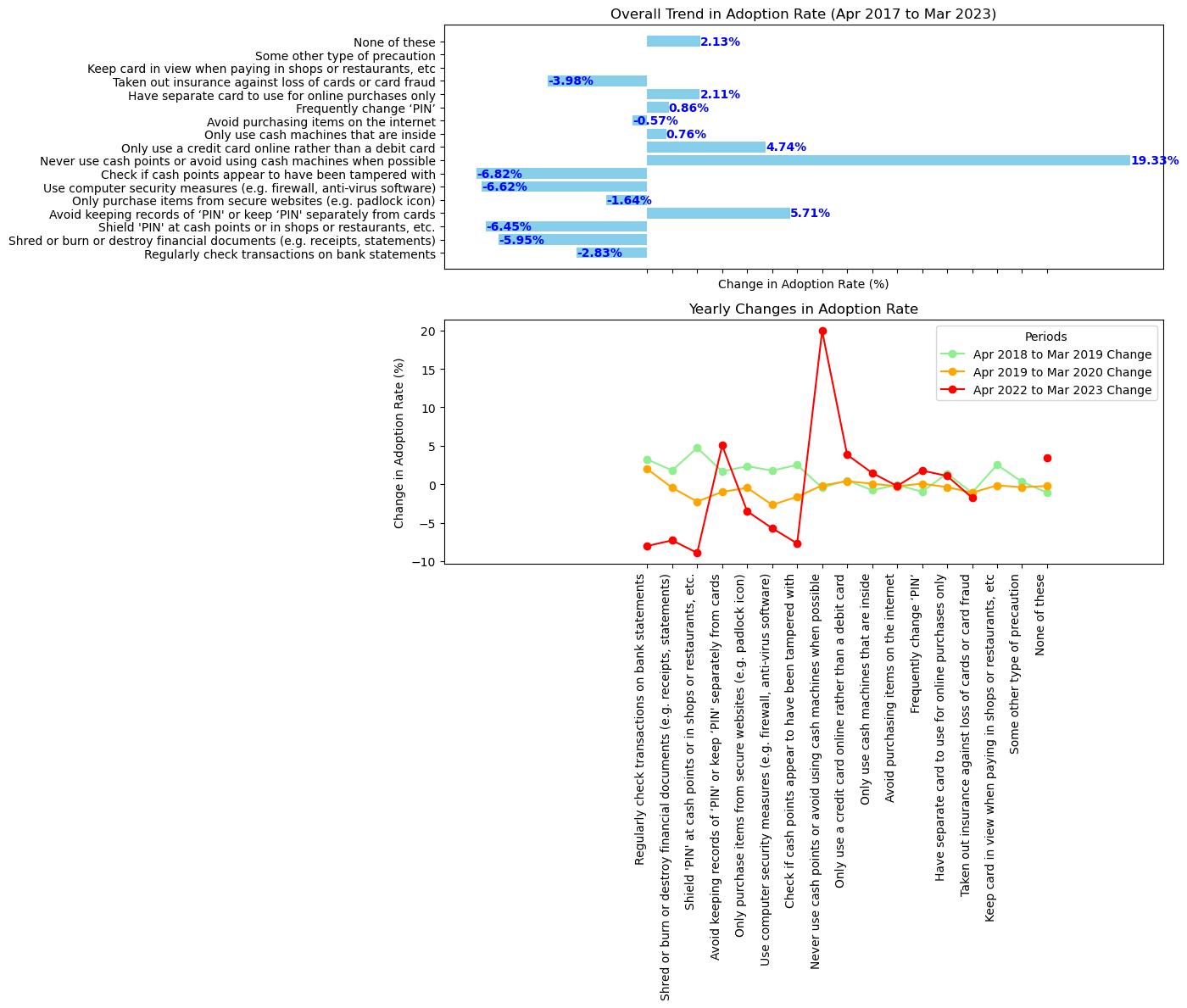
Adoption Rate Trend as a Whole from April 2017 to March 2023:
- The majority of preventative measures against card fraud or security issues have seen a decline in adoption over the past six years, as depicted in the bar chart.
- An exceptional circumstance is the recommendation to " Never use cash points or avoid using cash machines”, which experienced a substantial surge of around 19.33%. This behaviour may suggest an increased cognizance of the potential hazards linked to committing PINs to memory or carrying them with the card.
Diurnal Variations in the Adoption Rate:
- The line graph illustrates the annual fluctuations in the rates of adoption pertaining to each security measure.
- Adoption rates fluctuate between positive and negative trends annually across various metrics. Nonetheless, a general downward trend indicates that the implementation of the majority of these measures is diminishing in frequency.
- Numerous metrics exhibit a significant decline during the most recent period (April 2022 to March 2023).
As a result of these observations, the following future projections and implications are possible:
- Consumer complacency or reliance on unlisted security measures (e.g., technological advancements) may account for the overall decline in the implementation of the majority of security measures.
- The notable surge in the avoidance of PIN recording signifies that cardholders have successfully received and embraced this particular communication. This indicates that endeavours to educate the general public regarding this specific facet of card security are bearing fruit.
- Recent declines in the implementation of numerous measures may also be attributable to alterations in the payment ecosystem, including the proliferation of secure payment technologies (e.g., mobile wallets, contactless payments), which could render certain conventional measures obsolete.
- Close monitoring of these trends and undertaking additional research to ascertain the fundamental causes of these shifts are of utmost importance. Qualitative research has the potential to offer valuable insights regarding the determinants influencing consumer decisions regarding the adoption or rejection of specific security measures.
Method of Contact Victim
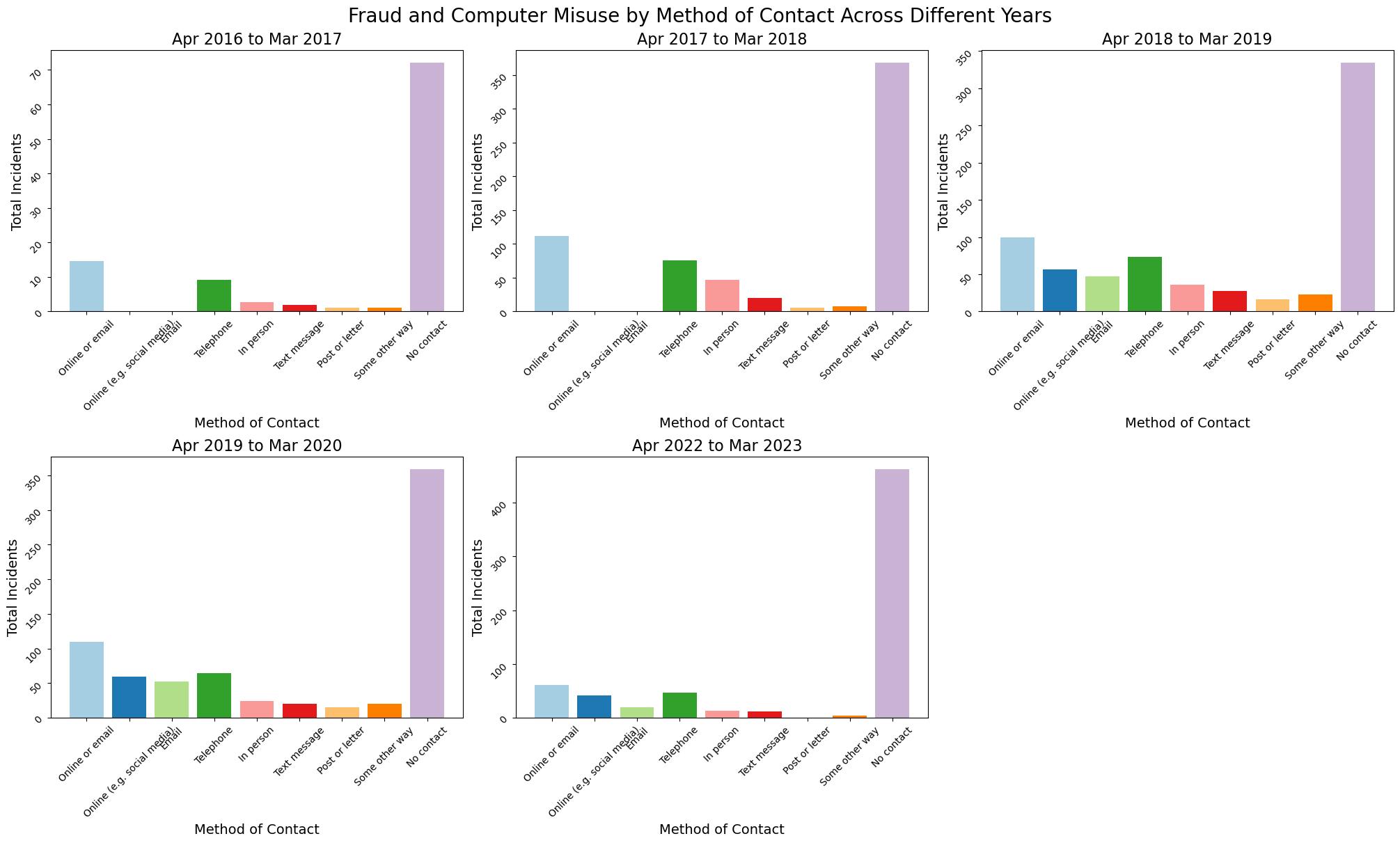
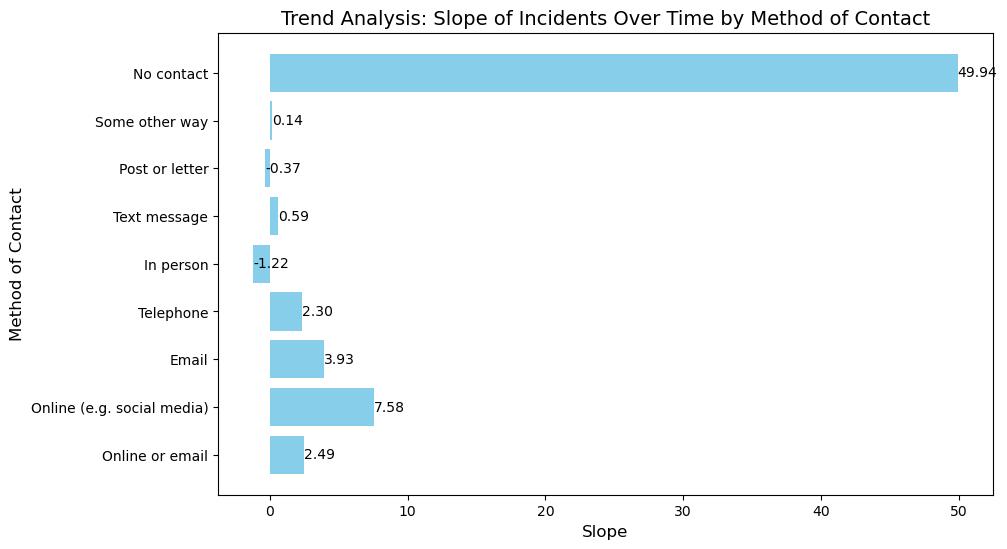
The table displays slopes and intercepts of a linear regression, explaining the changes in techniques used to get in touch with fraud victims in the period of 2016 to 2023. The positive gradient for "Online or email," "Online (e.g., social media)," and "Email" methods reveals that they are significantly utilized over time. The steepest increase is observed for social media (slope = 7.575494), a reflection of the increasing footprint of electronic channels on Internet fraud scams. The reverse situation is being reflected in the "In person" contact which has a negative slope (slope = -1.218619) maybe because people are now more interested in digital convenience or improved security measures. Surprisingly enough, "No contact" case has a very high slope (49.937251) which could mean that fraud activity, where victims have no clue, is increasing, such as in cases of identity theft or account takeovers. Telephone, text message, post or letter, and other undefined approaches have diverse patterns, yet the deeply rooted changes are rather minor compared to digital methods. Such transformation goes to show that cybersecurity is now more than a need, it is an essential part of a better digital world.
Reason for contact with offender
Trend Analysis: Chen et al. (2017) emphasizes that discrepancies between actual and predicted online behaviours can result from changing trends in Internet activities, which in turn affect victimization and privacy concerns. This is evident in the categories where actual values far exceed predictions, highlighting a possible underestimation of the rising trend in e-commerce activities and online scams, such as the categories "Buying or selling items online" and "Moving money out of bank account" (Chen, Beaudoin and Hong, 2017a).
Buying or Selling Items Online: Consistent with the upward trend in the "Buying or selling items online" category, research by Williams, shows that individual differences and contextual factors impact susceptibility to online influence, which can affect the frequency and nature of such transactions (Williams, Beardmore and Joinson, 2017). A more nuanced comprehension of online behaviours and the need for predictive models that are as dynamic and adaptable as the online environment itself are outcomes of the integration of these papers. As digital interactions progress, methodologies for comprehending, forecasting, and alleviating online risks must also evolve.
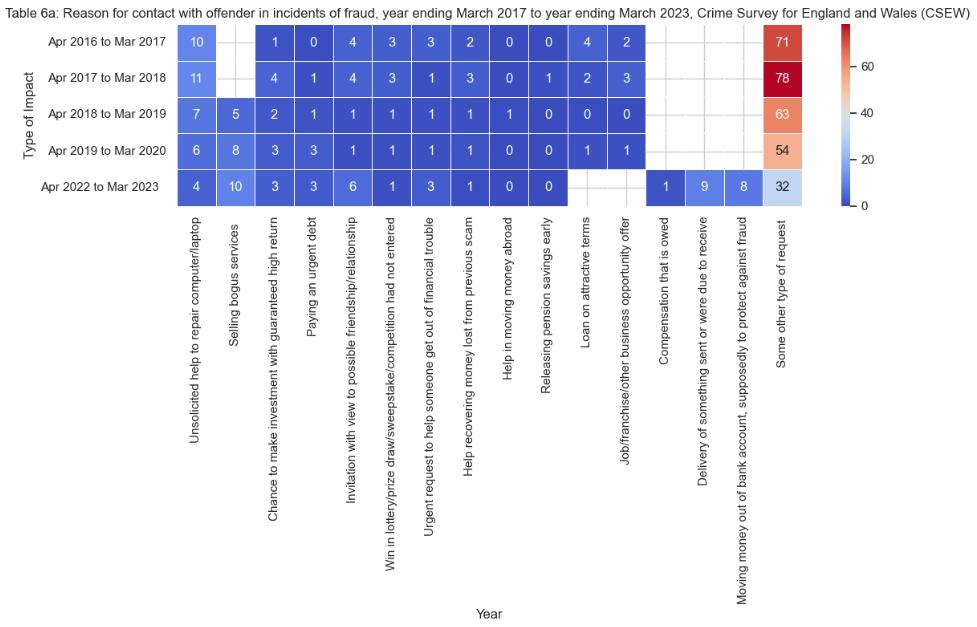
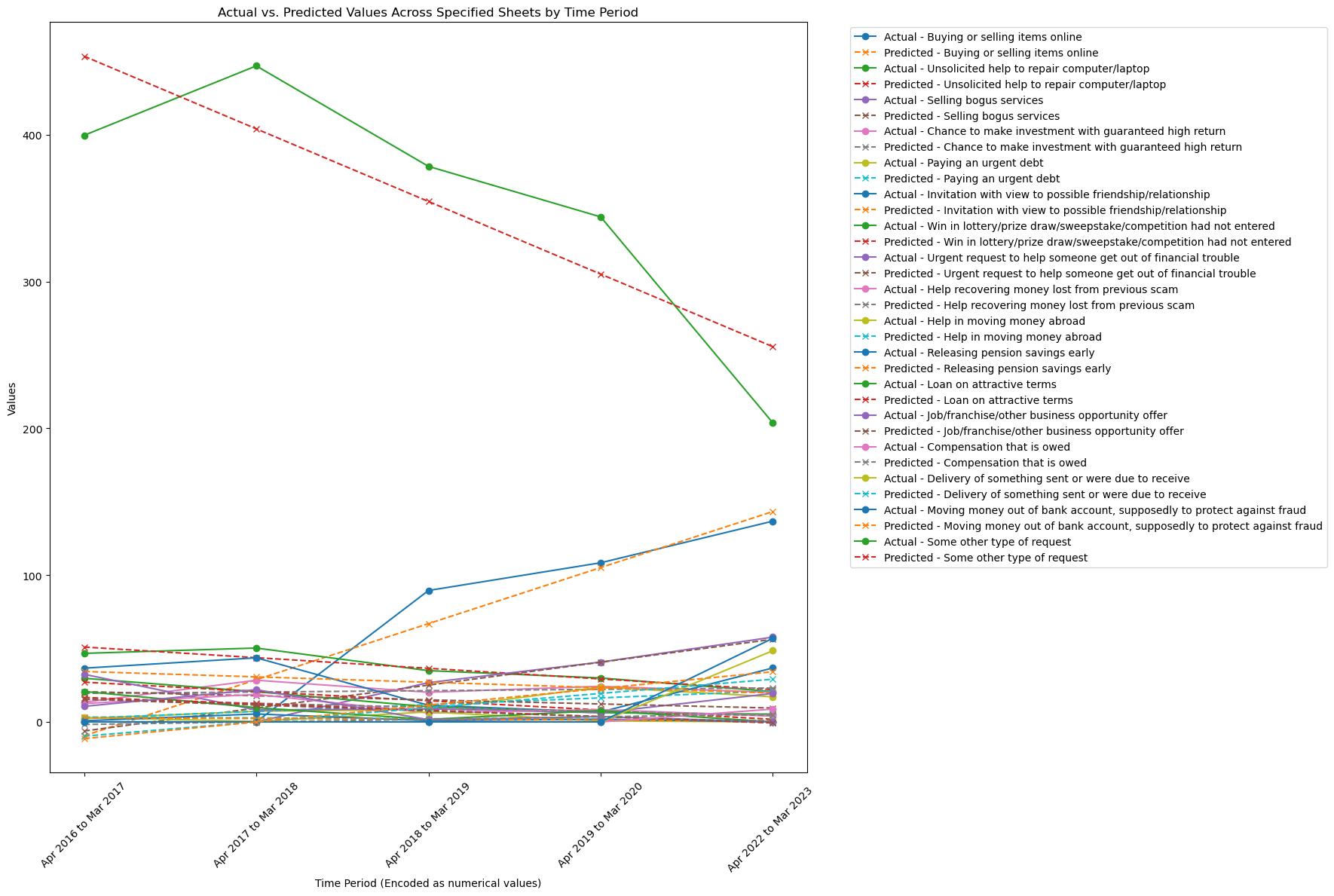
Gaining the access
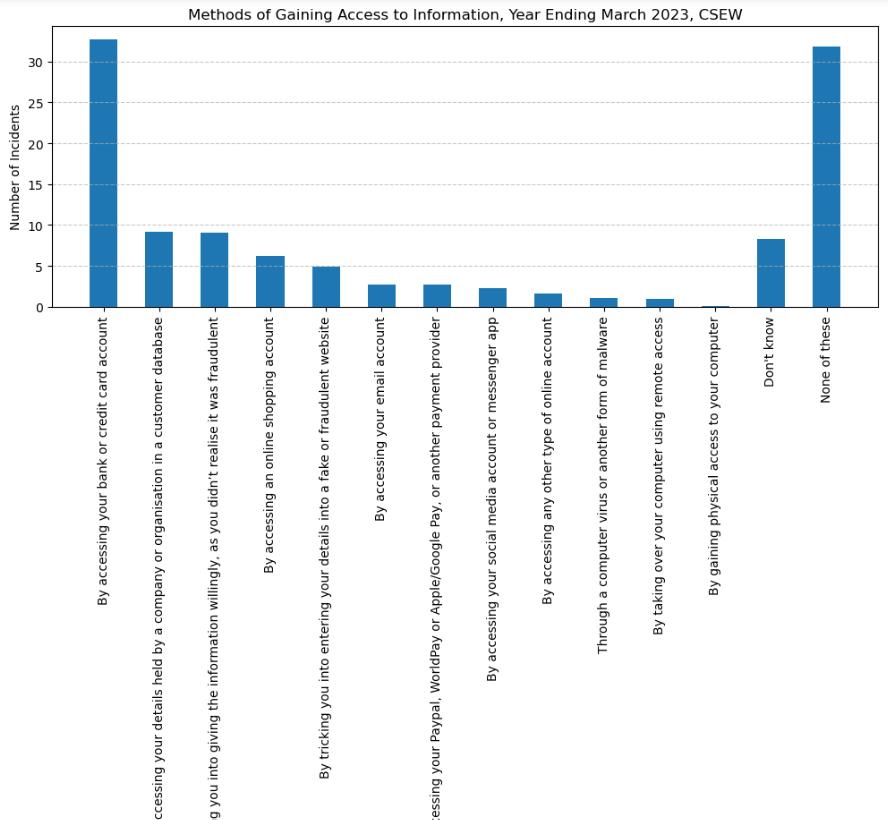
The data presented for the fiscal year ending in March 2023 indicates that the predominant means of obtaining information involved direct access to bank or credit card accounts. This was followed by breaches involving company or customer databases, as well as deceptive tactics aimed at coercing individuals into willingly divulging information. The continued significance of secure authentication processes and the necessity for vigilance against social engineering tactics are underscored by the prevalence of these methods. In the future, it is crucial to enhance cybersecurity protocols and raise public consciousness regarding the significance of safeguarding personal data, particularly as the integration of technology in daily transactions becomes more profound. It is imperative to closely observe patterns in malware and remote access exploits, which, although less common, pose substantial dangers because they can circumvent traditional. (Chen, Beaudoin and Hong, 2017b)
Action Fraud’s fraud incidents management
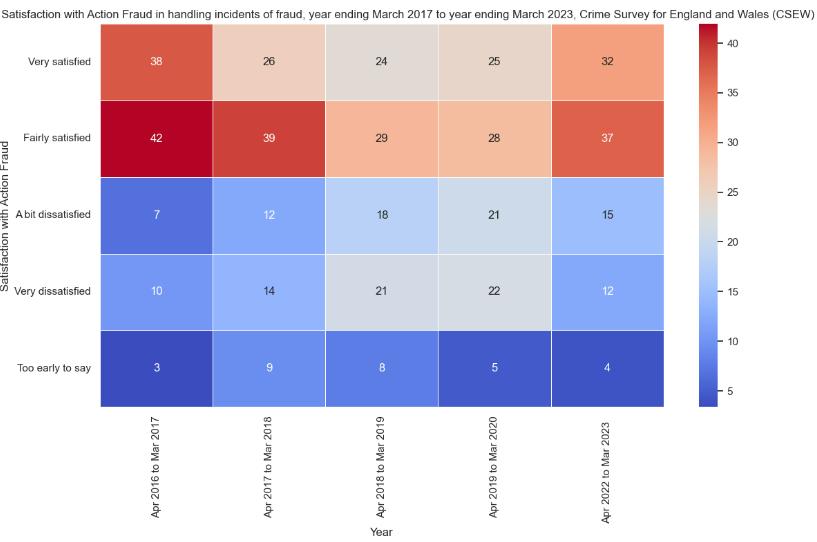
This heatmap shows how the population reacted to Action Fraud’s fraud incidents management in England and Wales from March, 2017 to March, 2023, as measured by the Crime Survey for England and Wales (CSEW). Redder colours mean more favourable number. Satisfaction seems to decrease with time. In the period 2016-2017, about 38% of people answered "very satisfied", and this percentage decreased notably to 32% in the period 2022-2023. "Satisficially" feedback also goes down. On the contrary, the level of dissatisfaction rises, where "Very dissatisfied" goes up from 10% to 22% over the same time. "Too early to say" category is at low level most of the time, signifying that most respondents were already able to form their opinion on this issue.
Emotional and Financial Impact of Frauds
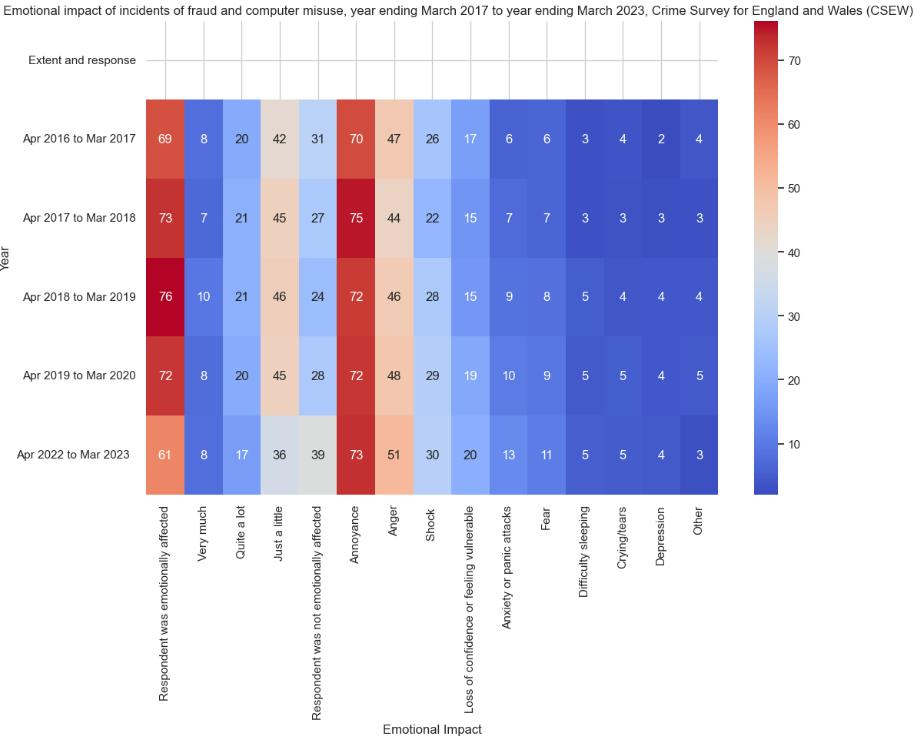
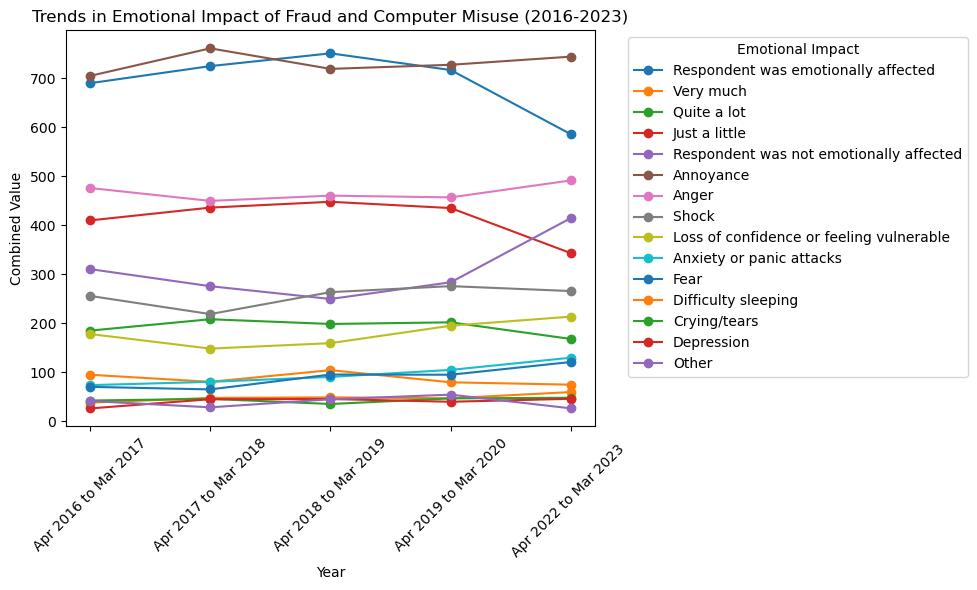
Examine the provided data and graphs and identify the subsequent patterns and their future ramifications:
The Analysis of Trends:
- Since April 2019 and March 2023, the overall emotional impact of fraud and computer misuse incidents on respondents has decreased substantially. Anticipate a significant decrease in emotional impact following a peak in April 2019.
- Emotional reactions such as 'Annoyance' and 'Anger' have experienced variations but continue to be relatively prevalent.
- In the most recent period of data collection (April 2022 to March 2023), the prevalence of 'Fear,' 'Loss of confidence or feeling vulnerable,' and 'Anxiety or panic attacks' has increased relative to the previous year. This suggests that while the overall number of respondents reporting emotional distress may be lower, those who do may be encountering more intense emotional reactions.
- Increased awareness, improved coping mechanisms, desensitization to such events, or a combination of these factors may account for the rise in the proportion of respondents who reported being "Not emotionally affected."
Future Implications:
- An ongoing decline in the overall count of individuals reporting emotional distress resulting from computer misuse and fraud is likely if the present pattern persists. However, among those affected, the severity of the effects may persist or even worsen.
- The higher percentage of participants who reported being unaffected emotionally may indicate a potential change in public opinion and consciousness, potentially attributable to enhanced security protocols, increased public awareness, or prolonged exposure to similar occurrences. This may also indicate that society is developing greater resilience to the psychological effects of cyber incidents.
The utilization of data-driven decision making:
- Further allocation of resources towards cybersecurity education and tools is probable due to the escalating prevalence of emotional reactions such as fear and anxiety, which serve as indicators of a persistent or growing perception of threat.
Considerations and Restrictions:
- It is imperative to comprehend the contextual underpinnings of the data, encompassing any modifications in reporting protocols, the incidence of fraudulent activities, or extraneous variables that could impact emotional reactions (e.g., a worldwide occurrence impacting emotional welfare).
- Anticipating forthcoming trends necessitates a prudent approach, given that they are susceptible to the vagaries of cyber threats, technological advancements, and societal transformations.
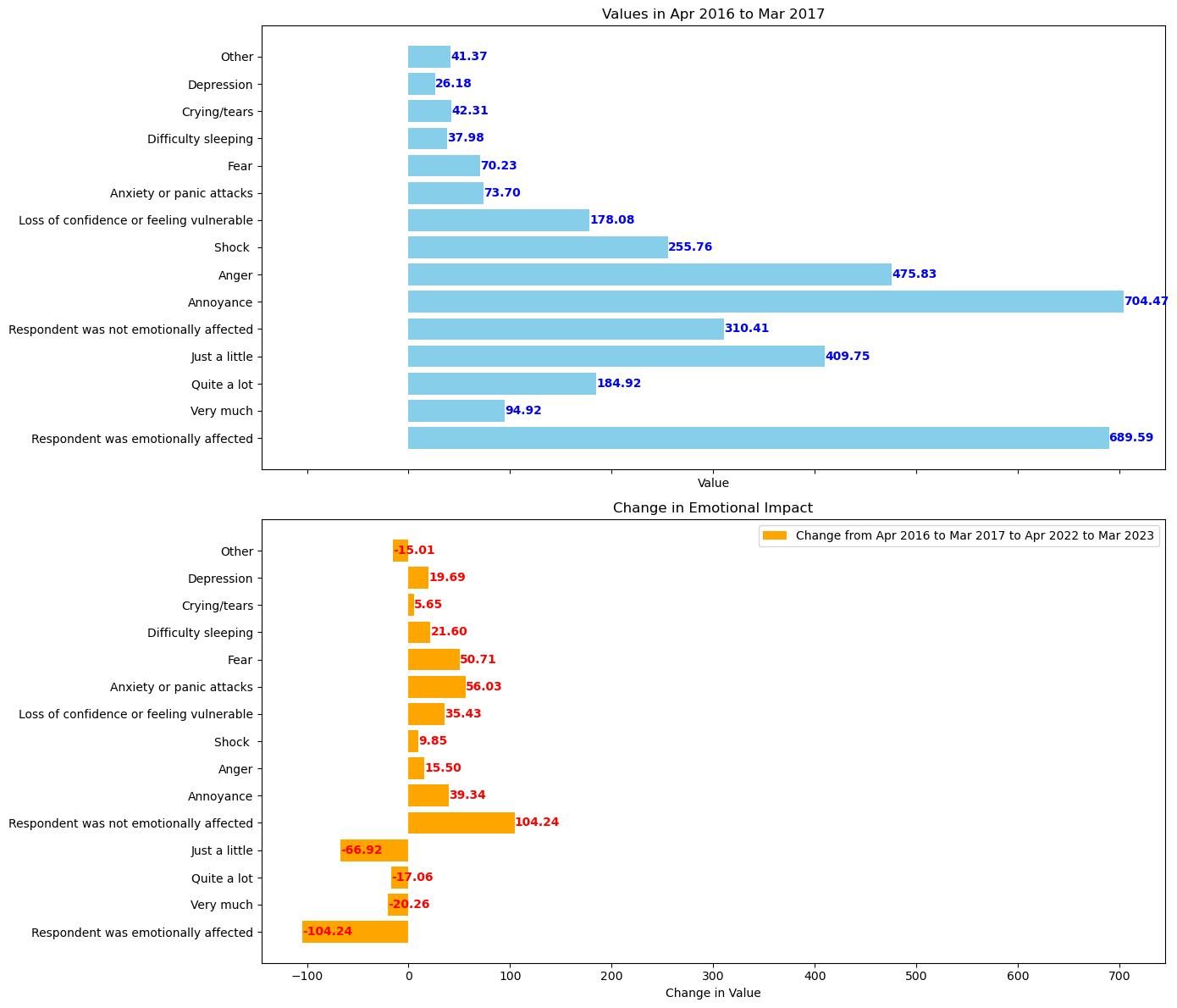
Reimbursement of money lost to victims
The chart and supplementary data present the proportions of victims who obtained reimbursement subsequent to fraudulent activities, categorized by offence type as general fraud, bank and credit account fraud, consumer and retail fraud, or general fraud.
Rate of Reimbursement:
The full reimbursement rate for victims of bank and credit account fraud is 84.22%, which is considerably higher than the rates of consumer and retail fraud (42.15%) and overall fraud (71.43%). The proportion of consumers and retailers who do not receive any reimbursement is the highest (49.24 percent), which is significantly higher than the rate of general fraud (21.05%) and considerably greater than that of bank and credit account fraud (8.99%). In all categories, the proportion of partial refunds is comparatively small, suggesting that reimbursements are generally all-or-nothing.
Unresolved Cases:
Among all types of fraud, the proportion of unresolved cases is comparatively modest. Specifically, consumer and retail fraud have the highest proportion (5.49%), while bank and credit account fraud have the lowest (3.98%).
Implications and Future Trends:
In light of the substantial reimbursement rates associated with credit and bank account fraud, this indicates that the banking industry has implemented robust consumer protection policies. Subsequent advancements in these policies and fraud detection systems may potentially result in increased reimbursement rates in the future.
The utilization of data-driven decision making:
In light of their current proficiency in customer refunds, financial institutions and banks may have every reason to uphold or enhance their protective measures. It may be necessary for retailers and consumer protection agencies to examine the strategies that banks employ in order to increase their reimbursement rates. Potentially enact stricter regulations on consumer and retail protections in an effort to reduce the number of non-refunded fraud cases by utilising the data to inform policy changes.
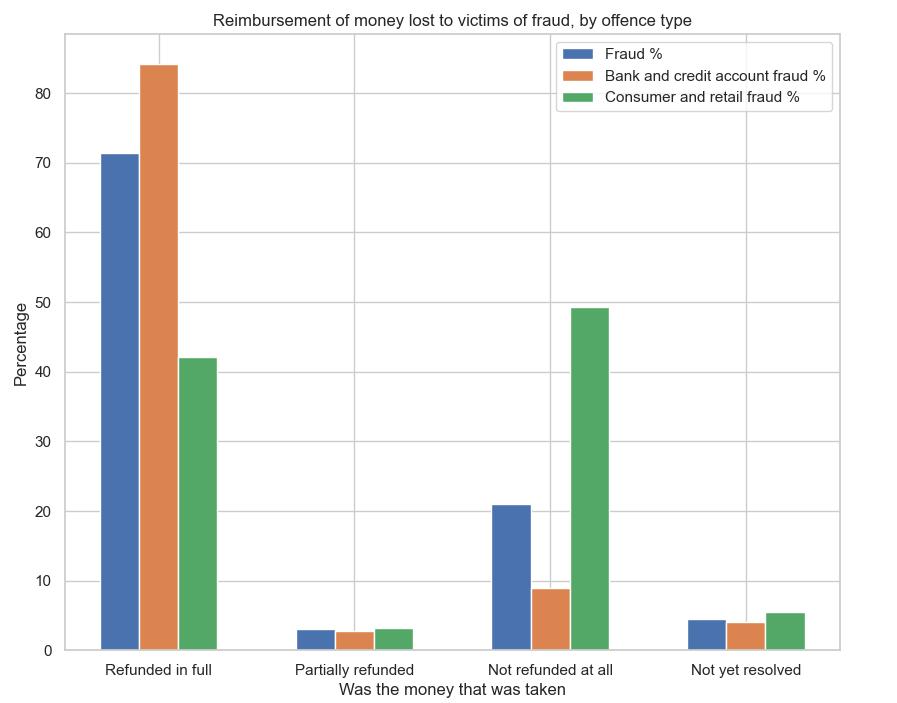
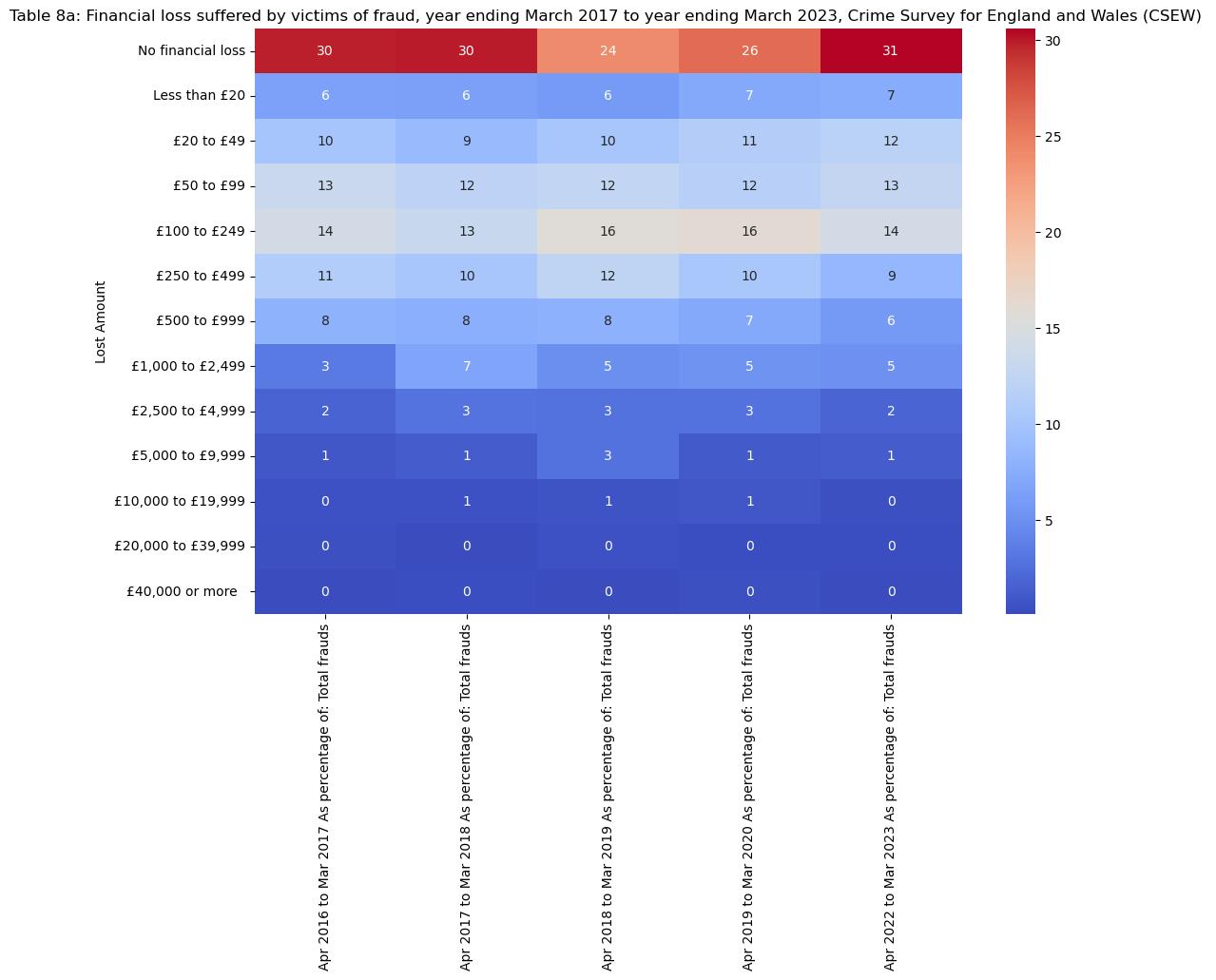
Financial loss suffered by victims of fraud
The literary work deals with a range of consequences of fraud victimization that are not exactly limited to the financial loss but also include the psychological and societal impacts. In accordance, the psychological consequence of fraud attacks can bring about a long-term effect on the confidence in money matters, which would affect the future financial decisions. (Brenner et al., 2020) These psychological aspects are confirmed by the studies regarding the victims' profiles and the advanced methods used by the fraudsters. The studies demonstrate that the online fraud schemes are not only pervasive but also sophisticated, therefore, they target trust and social connections for financial gains (Bar Lev, Maha and Topliceanu, 2022).
In addition, about the emotional and societal stigma that fraud victims have to bear, which is often regarded as their own fault or greed, and this further shows the need to eradicate the victim blaming and create a safe space for those affected (Joomratty, 2018). The examination of UK financial fraud including both empirical data and scholarly investigations indicates a multifaceted relationship among financial, psychological, and social issues. It emphasizes the importance of comprehensive strategies that include prevention, education, and victim support in order to mitigate the effects of financial fraud.
Number of frauds by police force area
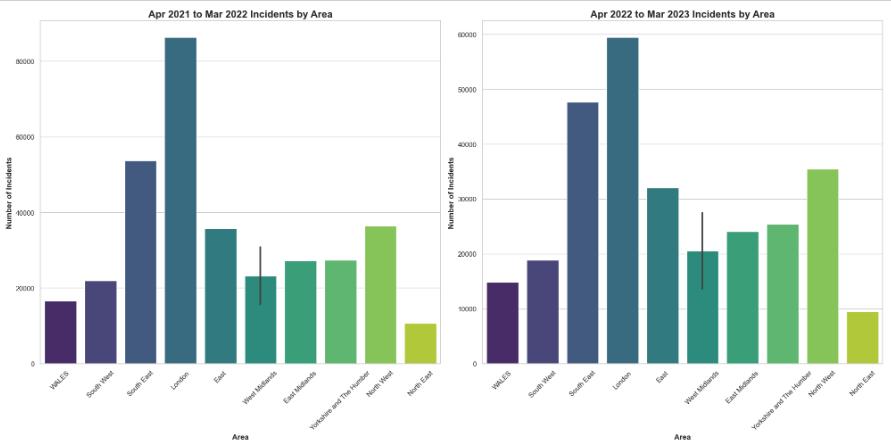
The bar charts illustrating the distribution of incidents across different regions over a span of two consecutive years demonstrate a general decline in incidents across all areas from April 2021 to March 2023. Notably, London exhibits the most substantial decrease in incidents during this period. The observed decline indicates the efficacy of existing measures aimed at preventing and intervening in cyber-fraud. In order to sustain this pattern, it will be necessary to consistently allocate resources towards cybersecurity, public education, and focused regional interventions, particularly in regions with higher per capita incident rates.(Whitty, 2019) These measures will help to further reduce these risks and safeguard the population.
Predictions
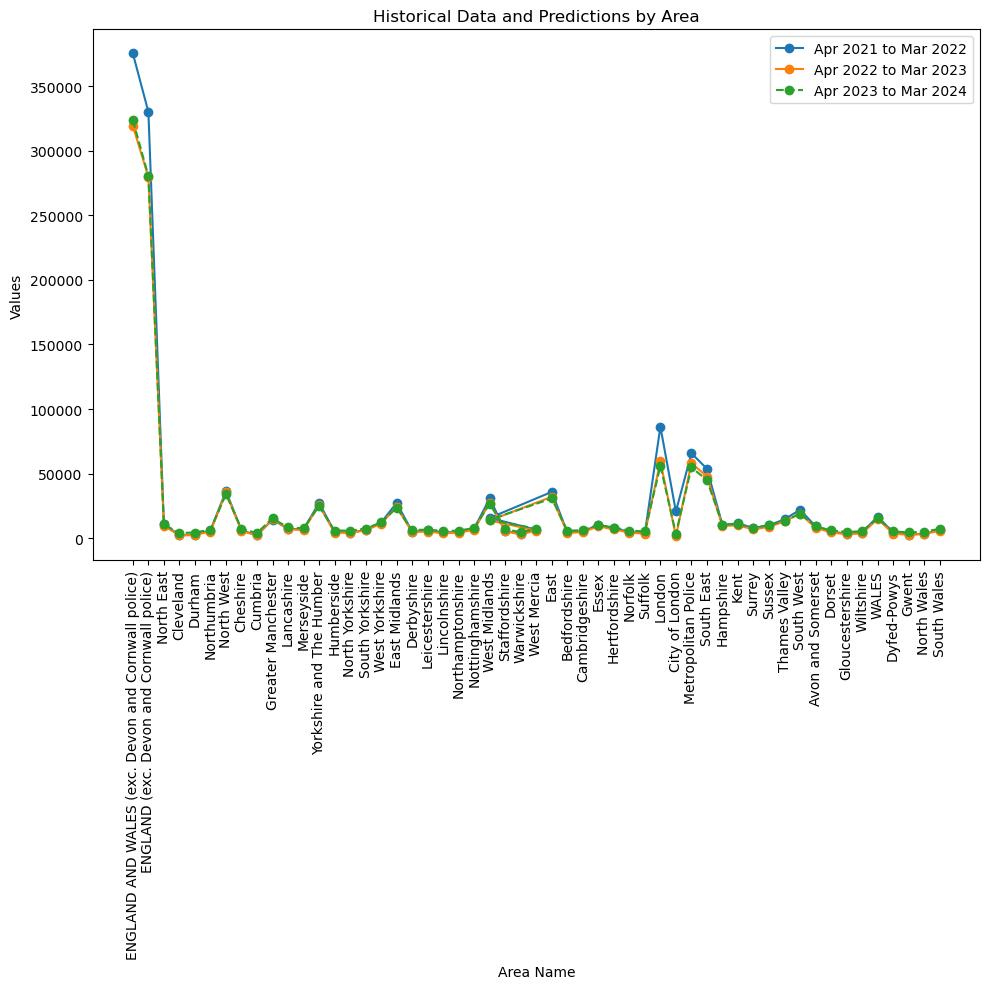
The LSTM model is highly advantageous for time-series forecasting as it can detect the long-term dependencies, and therefore is capable of generating more accurate predictions when compared to other models such as ARIMA for complex data patterns. The studies of Choi and Lee (2018) show that LSTM are models that provide a perceptible improvement of the forecasting accuracy with the ability to manage the nonlinearity and the temporal dynamics efficiently. Modelling process of LSTM involves preparation of data for normalization and building up a sequence-based LSTM structure, which corresponds LSTM's demonstrated advanced forecasting ability.(Choi and Lee, 2018),
In the Predictions, incident rates for the period from April 2023 to March 2024, as predicted, exhibit regional variation, with notable figures pertaining to England and Wales, and notably high figures observed in London and the Metropolitan Police areas. Consistent with the number of anticipated incidents, the forecasts emphasise the ongoing necessity for targeted cybersecurity interventions and measures in all regions. This underscores the significance of implementing focused strategies in order to further mitigate and efficiently control cyber-fraud risks.
Critical Analysis and Conclusion
The investigative journey into to address the SCAMS in the United Kingdom, as delineated by the comprehensive research and analysis conducted, underscores the acute vulnerability of the nation's financial ecosystem to a broad spectrum of fraudulent activities. This research has navigated through the multifaceted landscape of scams, leveraging advanced machine learning techniques and a keen understanding of demographic susceptibilities, geographical distributions, the temporal dynamics of fraudulent activities including Victims personal and household characteristic. this study not only aims to fulfil the objectives outlined in the initial research proposal but also propels forward a blueprint for predictive modelling and preventative strategies that align with the intricate nature of scam methodologies and the ethical boundaries within which they must operate. Report clearly shown that the SCAM detection application using SVM models, SARIMA and ARIMA models has proved effective in flagging potential scam emails, demonstrating the role of machine learning in the prevention of fraud.
Summary of Key Findings and Theoretical Contributions
- Temporal and Geographical Insights: The recognition of certain patterns and trends among different regions and timeframes within the UK shows the complex nature of scam activities that lies at the heart of the implementation of targeted preventive measures and the creation of predictable models of risk management.
- Demographic Vulnerabilities: The study in this area points out the group of people who are more likely to fall for scams. The study also helps one to see the role of age, location, gender and the household characteristics in the vulnerability to fraud. This segmentation is critical in the sense that it allows for the development of tailored solutions and for creating protective measures.
- Machine Learning Advancements: The research is mainly conducted with the use of machine learning methods for the identification and categorization of fraud. This process, wherein the model underperformance is studied to learn the capabilities and challenges in using AI for fraud detection and categorization, underlines the power and limitations with such an approach.
- Ethical and Legal Frameworks: The integration of ethical and legal considerations in the predictive models illustrates researcher's aim of not only advancing technological capabilities but also ensuring that advancements are done in a right and legal way which safeguard privacy as well as fairness.
- Comprehensive Preventative Measures: The strategy proposed for scam prevention in UK which is based on the complexity of the scam ecosystem, is ample evidence of the depth of thinking towards the issue. It is based on collective effort, incorporating technology, regulation and consciousness as the backbone of the defines against fraud.
The present study extended the initial proposal and analysed scams from a wide perspective, including the personal and financial aspects that the victims have to face. The human element is thus the missing piece of the technical and data-driven puzzle, which are the foundations of the study. By proposing solutions that are mindful of the technical complexities and human nature, these solutions will be holistic and effective.
Implications for Future Research
- Data Quality Enhancement: Attempts in the future should be concentrated on building varied, accurate and reliable datasets that will represent the dynamic nature of the scams, increasing the sophistication of models and their resistance to errors.
- Interdisciplinary Collaboration: This can be achieved through conversations with psychologists, criminologists, and lawyers and will help to provide more complete information and smarter predictive models.
- Advanced Machine Learning Techniques: The use of state-of-the-art approaches such as deep learning and natural language processing could help in revealing new scam patterns and that could be the new breakthrough in scam detections.
- Real-time Detection Systems: The real-time fraud detection systems development is the next crucial step that can not only prevent scams, but also react to new tricks at the moment they emerge. For example, the law enforcement can find the threats and area quickly so they can take action quickly.
- Ethical AI and Transparency: Guaranteeing that predictive models are in line with ethical standards and transparency is a vital point, which in turn shows that explainable AI is of great importance since it contributes to people's trust and accountability.
Conclusion
The completion of research on online fraud in the United Kingdom has successfully addressed key questions and met its stated goals. This body of work has made significant contributions to the understanding and combating of fraudulent activities by focusing on the temporal and geographical patterns of fraud, the impact of demographic factors on scam vulnerability, the efficacy of machine learning in fraud prediction and classification, and the importance of ethical and legal considerations in model development. Significant progress has been made as a result of the implementation of a scam detection platform, a thorough review of relevant literature, and the conduct of empirical studies. Notably, incorporating artificial intelligence (AI) into cybersecurity measures has been identified as a critical step towards improving scam detection and prevention efforts (Reynolds et al., 2019). The findings of this comprehensive study emphasise the importance of continuing to invest in both educational initiatives and technological innovations in order to effectively combat online fraud. The development and testing of a SPAM email detection system demonstrate the power of technology to overcome the challenges posed by scams. Furthermore, detailed investigations into fraudulent schemes and victim profiling have highlighted the critical need for targeted preventive measures and security protocols. A comprehensive approach that combines technological solutions, increased public awareness, and stringent legal regulations is required to protect individuals and communities from the widespread financial and psychological consequences of fraud. Given the ever-changing landscape of cyber threats, there is ample opportunity for future research to broaden the existing knowledge base and investigate novel methods for improving internet security and fraud prevention. This study emphasises the importance of ongoing exploration and adaptation in addressing the complex dynamics of online scams, highlighting the critical role of relentless innovation and scholarly inquiry in this domain.
8. References
Abadi, A., Murdoch, S.J. and Zacharias, T. (2023) ‘Recurring Contingent Service Payment’, Proceedings - 8th IEEE European Symposium on Security and Privacy, Euro S and P 2023, pp. 724–756. Available at: https://doi.org/10.1109/EUROSP57164.2023.00049.
Abbott, R.D. (1985) ‘LOGISTIC REGRESSION IN SURVIVAL ANALYSIS’, American Journal of Epidemiology, 121(3), pp. 465–471. Available at: https://doi.org/10.1093/OXFORDJOURNALS.AJE.A114019.
Action Fraud . Available at: https://www.actionfraud.police.uk/ (Accessed: 21 March 2024).
Alnajim, A. and Munro, M. (2009) ‘An anti-phishing approach that uses training intervention for phishing websites detection’, ITNG 2009 - 6th International Conference on Information Technology: New Generations, pp. 405–410. Available at: https://doi.org/10.1109/ITNG.2009.109.
Al-Rousan, S. et al. (2020) ‘Ads-Guard: Detecting Scammers in Online Classified Ads’, 2020 IEEE Symposium Series on Computational Intelligence, SSCI 2020, pp. 1492–1498. Available at: https://doi.org/10.1109/SSCI47803.2020.9308544.
Annual Fraud Report 2022 | Policy and Guidance | UK Finance . Available at: https://www.ukfinance.org.uk/policy-and-guidance/reports-and-publications/annual-fraud-report-2022 (Accessed: 15 February 2024).
Bainbridge, D. (2007) ‘Criminal law tackles computer fraud and misuse’, Computer Law and Security Review, 23(3), pp. 276–281. Available at: https://doi.org/10.1016/J.CLSR.2007.03.001.
Bains, K. et al. (2021) ‘Defeating the Credit Card Scams through Machine Learning Algorithms’, 2021 International Conference on Innovation and Intelligence for Informatics, Computing, and Technologies, 3ICT 2021, pp. 193–198. Available at: https://doi.org/10.1109/3ICT53449.2021.9582060.
Bandyopadhyay, S. ‘Detection of Fraud Transactions Using Recurrent Neural Network during COVID-19’, Journal of Advanced Research in Medical Science & Technology [Preprint]. Available at: https://doi.org/10.20944/PREPRINTS202006.0368.V1.
Bar Lev, E., Maha, L.G. and Topliceanu, S.C. (2022) ‘Financial frauds’ victim profiles in developing countries’, Frontiers in psychology, 13. Available at: https://doi.org/10.3389/FPSYG.2022.999053.
Brenner, L. et al. (2020) ‘Consumer fraud victimization and financial well-being’, Journal of Economic Psychology, 76. Available at: https://doi.org/10.1016/J.JOEP.2019.102243.
Buil-Gil, D. et al. (2020) ‘Cybercrime and shifts in opportunities during COVID-19: a preliminary analysis in the UK’, European Societies: The Official Journal of the European Sociological Association, 23(S1), pp. S47–S59. Available at: https://doi.org/10.1080/14616696.2020.1804973.
Buil-Gil, D. et al. (2021a) ‘Cybercrime and shifts in opportunities during COVID-19: a preliminary analysis in the UK’, European Societies, 23(S1), pp. S47–S59. Available at: https://doi.org/10.1080/14616696.2020.1804973.
Buil-Gil, D. et al. (2021b) ‘Cybercrime and shifts in opportunities during COVID-19: a preliminary analysis in the UK’, European Societies, 23(S1), pp. S47–S59. Available at: https://doi.org/10.1080/14616696.2020.1804973.
Chen, H., Beaudoin, C.E. and Hong, T. (2017a) ‘Securing online privacy: An empirical test on Internet scam victimization, online privacy concerns, and privacy protection behaviors’, Computers in Human Behavior, 70, pp. 291–302. Available at: https://doi.org/10.1016/J.CHB.2017.01.003.
Chen, H., Beaudoin, C.E. and Hong, T. (2017b) ‘Securing online privacy: An empirical test on Internet scam victimization, online privacy concerns, and privacy protection behaviors’, Computers in Human Behavior, 70, pp. 291–302. Available at: https://doi.org/10.1016/J.CHB.2017.01.003.
Choi, J.Y. and Lee, B. (2018) ‘Combining LSTM Network Ensemble via Adaptive Weighting for Improved Time Series Forecasting’, Mathematical Problems in Engineering, 2018. Available at: https://doi.org/10.1155/2018/2470171.
Close-Up: Q - Do scam ads pose a problem in the UK? - ProQuest . Available at: https://www.proquest.com/docview/1372757416?parentSessionId=FmU1qMTK72nK7FaWIkowUXYiFk%2BFI8Oqe%2FWjTAcopW4%3D&accountid=27114&sourcetype=Trade%20Journals (Accessed: 9 March 2024).
Coluccia, A. et al. (2020a) ‘Online Romance Scams: Relational Dynamics and Psychological Characteristics of the Victims and Scammers. A Scoping Review’, Clinical Practice and Epidemiology in Mental Health, 16(1), pp. 24–35. Available at: https://doi.org/10.2174/1745017902016010024.
Coluccia, A. et al. (2020b) ‘Online Romance Scams: Relational Dynamics and Psychological Characteristics of the Victims and Scammers. A Scoping Review’, Clinical Practice and Epidemiology in Mental Health, 16(1), pp. 24–35. Available at: https://doi.org/10.2174/1745017902016010024.
Correia, S.G. (2019) ‘Responding to victimisation in a digital world: a case study of fraud and computer misuse reported in Wales’, Crime Science, 8(1). Available at: https://doi.org/10.1186/S40163-019-0099-7.
Dahlgreen, J. (2023) ‘Catastrophic fraud loss lies where it falls? Push payment scams and the bank’s duty of care to its customer’, Journal of Financial Crime, 30(6), pp. 1845–1852. Available at: https://doi.org/10.1108/JFC-10-2021-0223/FULL/XML.
Dong, Y., Li, S. and Gong, X. (2017) ‘Time Series Analysis: An application of ARIMA model in stock price forecasting’. Available at: https://doi.org/10.2991/IEMSS-17.2017.140.
Eost-Telling, C.L. et al. (2019) ‘The methodological relevance of mass observation data’, Innovation in Aging, 3(Supplement_1), pp. S757–S757. Available at: https://doi.org/10.1093/GERONI/IGZ038.2778.
Faith, B., Hernandez, K. and Beecher, J. ‘Digital poverty sets up a vicious circle: those without digital connectivity lose out and become financially poorer. Digital Poverty in the UK’.
‘FRAUD-THE FACTS 2020 The definitive overview of payment industry fraud’ .
Hunter, J.D. (2007) ‘Matplotlib: A 2D graphics environment’, Computing in Science and Engineering, 9(3), pp. 90–95. Available at: https://doi.org/10.1109/MCSE.2007.55.
Joomratty, Q.B. (2018) ‘“STRIPPED WALLETS, RIPPED HEARTS” VICTIMS OF FINANCIAL FRAUD: AN ANALYSIS BEYOND FINANCIAL FRAUD’, PEOPLE: International Journal of Social Sciences, 4(3), pp. 1101–1112. Available at: https://doi.org/10.20319/PIJSS.2019.43.11011112.
Josyula, H.P., Vishnubhotla, D. and Onyando, P.O. (2023) ‘Is Artificial Intelligence an Efficient Technology for Financial Fraud Risk Management?’, www.arcjournals.org International Journal of Managerial Studies and Research, 11(6), pp. 11–16. Available at: https://doi.org/10.20431/2349-0349.1106002.
Kaur, R., Gabrijelčič, D. and Klobučar, T. (2023) ‘Artificial intelligence for cybersecurity: Literature review and future research directions’, Information Fusion, 97. Available at: https://doi.org/10.1016/J.INFFUS.2023.101804.
Kemp, S. et al. (2021) ‘Empty Streets, Busy Internet: A Time-Series Analysis of Cybercrime and Fraud Trends During COVID-19’, Journal of Contemporary Criminal Justice, 37(4), pp. 480–501. Available at: https://doi.org/10.1177/10439862211027986/ASSET/IMAGES/LARGE/10.1177_10439862211027986-FIG5.JPEG.
Larson, J. et al. (2018) ‘Using Web-Scale Graph Analytics to Counter Technical Support Scams’, Proceedings - 2018 IEEE International Conference on Big Data, Big Data 2018, pp. 3968–3971. Available at: https://doi.org/10.1109/BIGDATA.2018.8621871.
Lee, N.M. (2018) ‘Fake news, phishing, and fraud: a call for research on digital media literacy education beyond the classroom’, Communication Education, 67(4), pp. 460–466. Available at: https://doi.org/10.1080/03634523.2018.1503313.
Licences - Office for National Statistics . Available at: https://www.ons.gov.uk/methodology/geography/licences (Accessed: 19 March 2024).
Liu, C. et al. (2016) ‘Online ARIMA Algorithms for Time Series Prediction’, AAAI Conference on Artificial Intelligence, pp. 1867–1873. Available at: https://doi.org/10.1609/AAAI.V30I1.10257.
Ma, K.W.F., Dhot, T. and Raza, M. (2023) ‘Considerations for Using Artificial Intelligence to Manage Authorized Push Payment (APP) Scams’, IEEE Engineering Management Review, 51(3), pp. 166–179. Available at: https://doi.org/10.1109/EMR.2023.3288432.
Mckinney, W. (2010) ‘Data Structures for Statistical Computing in Python’.
McKinney, W. ‘Python for Data Analysis’. Available at: www.it-ebooks.info (Accessed: 20 March 2024).
Murray, A. (2019) ‘19. Computer misuse’, Information Technology Law, pp. 473–503. Available at: https://doi.org/10.1093/HE/9780198804727.003.0019.
National Fraud Initiative Report: December 2022 (HTML) - GOV.UK . Available at: https://www.gov.uk/government/publications/national-fraud-initiative-reports/national-fraud-initiative-report-december-2022-html (Accessed: 14 February 2024).
Nature of fraud and computer misuse in England and Wales Articles - Office for National Statistics . Available at: https://www.ons.gov.uk/peoplepopulationandcommunity/crimeandjustice/articles/natureoffraudandcomputermisuseinenglandandwales/previousReleases (Accessed: 5 March 2024).
Norris, G. and Brookes, A. (2021) ‘Personality, emotion and individual differences in response to online fraud’, Personality and Individual Differences, 169, p. 109847. Available at: https://doi.org/https://doi.org/10.1016/j.paid.2020.109847.
Pedregosa, F. et al. (2012) ‘Scikit-learn: Machine Learning in Python’. Available at: http://arxiv.org/abs/1201.0490 (Accessed: 20 March 2024).
Pedregosa FABIANPEDREGOSA, F. et al. (2011) ‘Scikit-learn: Machine Learning in Python Gaël Varoquaux Bertrand Thirion Vincent Dubourg Alexandre Passos PEDREGOSA, VAROQUAUX, GRAMFORT ET AL. Matthieu Perrot’, Journal of Machine Learning Research, 12, pp. 2825–2830. Available at: http://scikit-learn.sourceforge.net. (Accessed: 19 March 2024).
Quality and methodology information - Office for National Statistics . Available at: https://www.ons.gov.uk/methodology/methodologytopicsandstatisticalconcepts/qualityinofficialstatistics/qualityandmethodologyinformation (Accessed: 19 March 2024).
Ranganathan, P., Pramesh, C. and Aggarwal, R. (2017) ‘Common pitfalls in statistical analysis: Logistic regression’, Perspectives in clinical research, 8(3), pp. 148–151. Available at: https://doi.org/10.4103/PICR.PICR_87_17.
Rosiene, J.A. and Pe Rosiene, C. (2020) ‘SPAM: Simplifying Python for Approaching Machine Learning’, Proceedings - Frontiers in Education Conference, FIE, 2020-October. Available at: https://doi.org/10.1109/FIE44824.2020.9273972.
Seabold, S. and Perktold, J. (2010) ‘Statsmodels: Econometric and Statistical Modeling with Python’, SciPy, pp. 92–96. Available at: https://doi.org/10.25080/MAJORA-92BF1922-011.
Sokolova, M. and Lapalme, G. (2009) ‘A systematic analysis of performance measures for classification tasks’, Information Processing & Management, 45(4), pp. 427–437. Available at: https://doi.org/10.1016/J.IPM.2009.03.002.
Spam, scam, scam, scam - ProQuest . Available at: https://www.proquest.com/docview/2523075292?parentSessionId=tUsxNCcbLgo4l7sBvxnLH%2FXZqsbg%2FXU1vJXGiAQ8Fjs%3D&accountid=27114&sourcetype=Magazines (Accessed: 9 March 2024).
UK Finance: Cross-sector cooperation needed to tackle rise in authorised push payment fraud | Insights | UK Finance . Available at: https://www.ukfinance.org.uk/uk-finance-cross-sector-cooperation-needed-tackle-rise-authorised-push-payment-fraud (Accessed: 10 March 2024).
Uk, U.O. ‘2020 HALF YEAR FRAUD UPDATE’.
Whitty, M.T. (2019) ‘Predicting susceptibility to cyber-fraud victimhood’, Journal of Financial Crime, 26(1), pp. 277–292. Available at: https://doi.org/10.1108/JFC-10-2017-0095.
Whitty, M.T. and Buchanan, T. (2012) ‘The Online Romance Scam: A Serious Cybercrime’, Cyberpsychology, behavior and social networking, 15 3(3), pp. 181–3. Available at: https://doi.org/10.1089/CYBER.2011.0352.
Williams, E.J., Beardmore, A. and Joinson, A.N. (2017) ‘Individual differences in susceptibility to online influence: A theoretical review’, Computers in Human Behavior, 72, pp. 412–421. Available at: https://doi.org/10.1016/J.CHB.2017.03.002.
Xia, P. et al. (2020) ‘Characterizing Cryptocurrency Exchange Scams’, Computers & security, 98. Available at: https://doi.org/10.1016/J.COSE.2020.101993.
Yang, L. and Qiuxiang, Z. (2022) ‘Research on E-Commerce User Interest Recommendation Method Based on TF-IDF Algorithm’, Proceedings - 2022 2nd Asia-Pacific Conference on Communications Technology and Computer Science, ACCTCS 2022, pp. 291–295. Available at: https://doi.org/10.1109/ACCTCS53867.2022.00066.
Appendices
SCAM Email detector Web base App “app.py” file:
IDE - Visual Studio Code
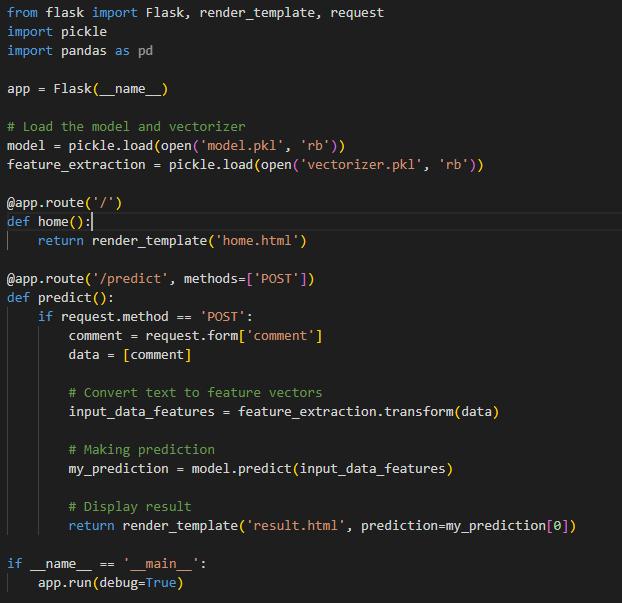
train_model.py file
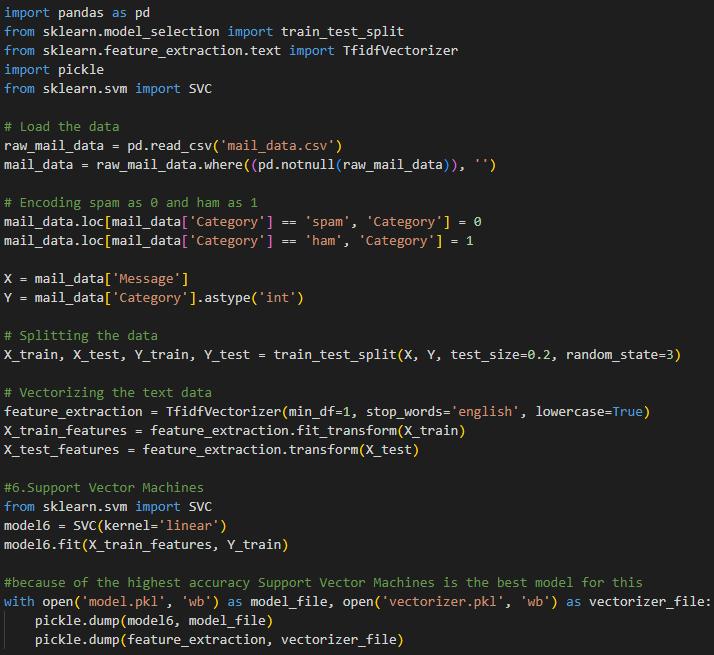
File Structure
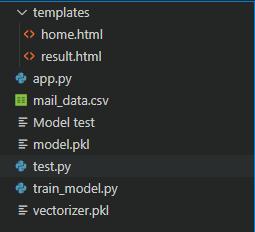
Dataset Analysis
SARIMAX model Training : IDE - Visual Studio Code
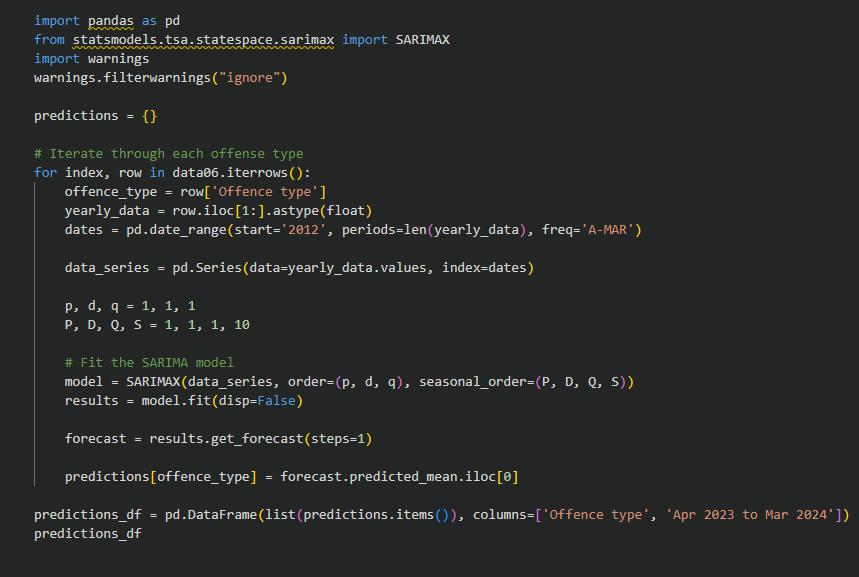
ARIMA model
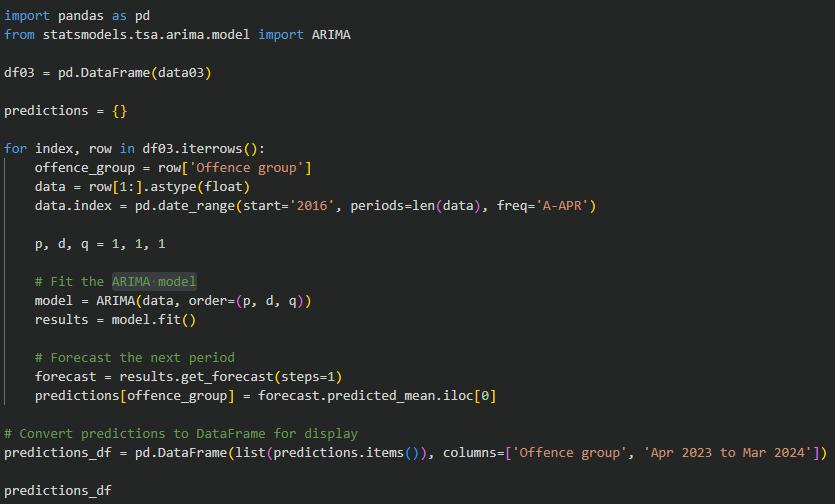
Sampel of Heat map visualization
IDE - Jupyter Notebook
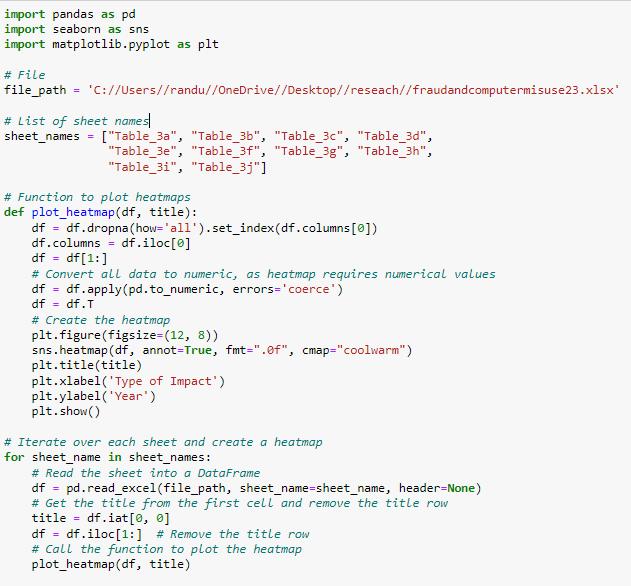
Sample of a plot used to visualized the changes over the years;
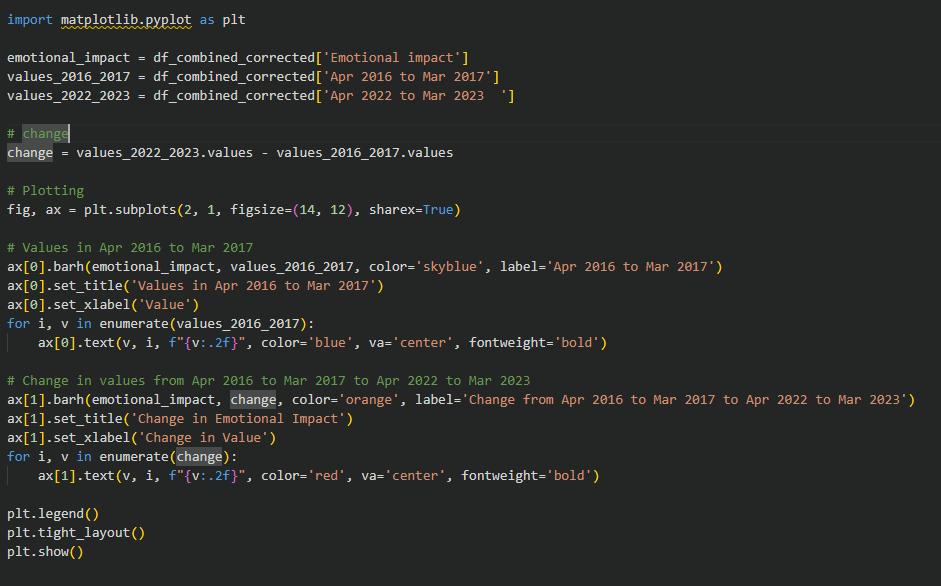
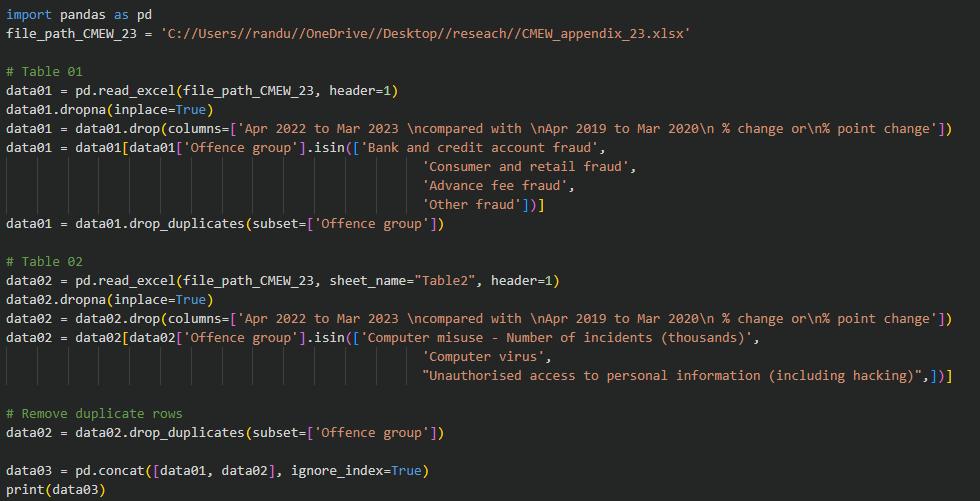
Visualizations of the Findings
Selected Trends in Impacts of Fraud and Computer Misuse (2017-2023)
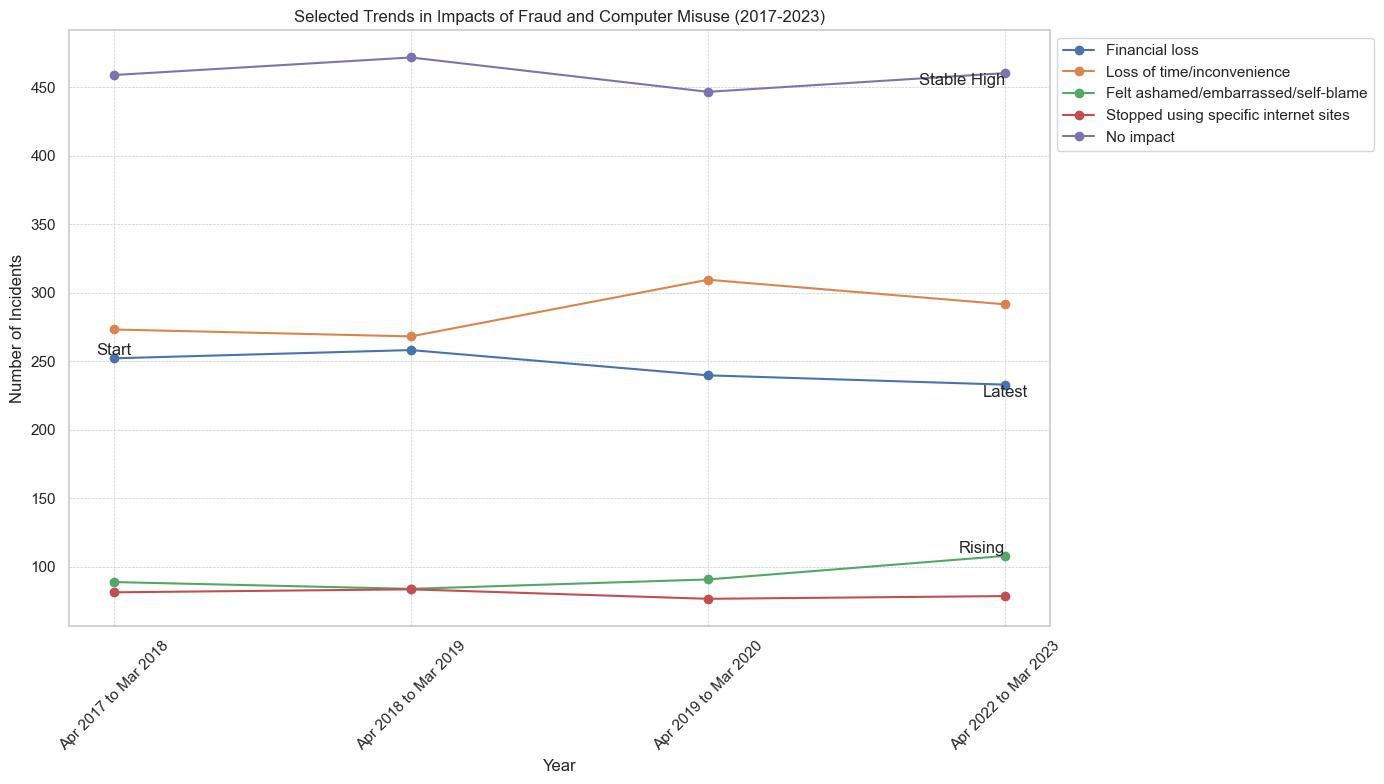
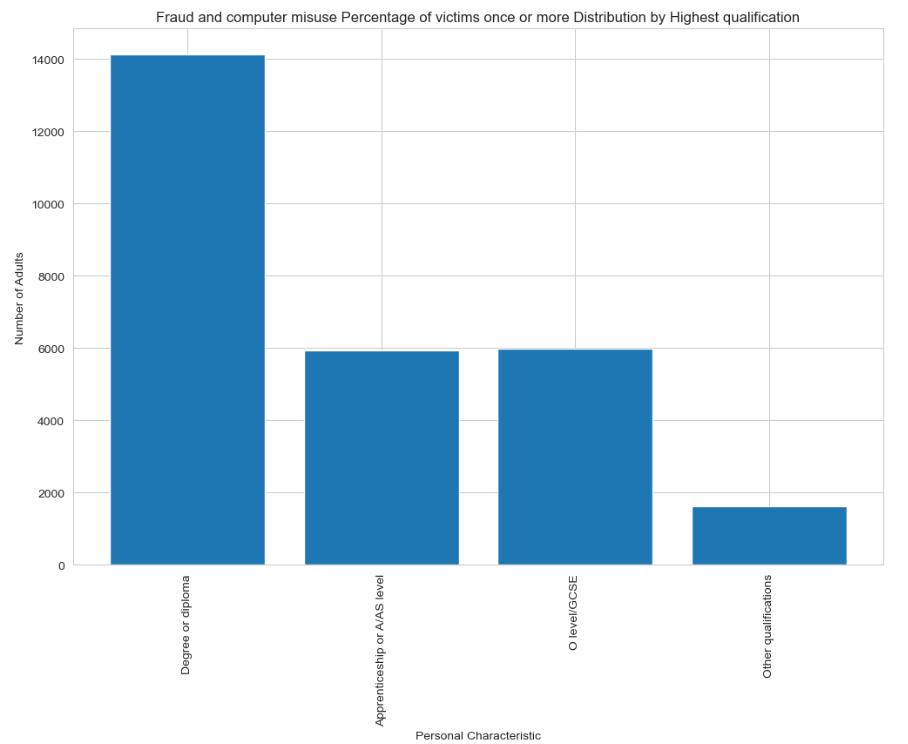
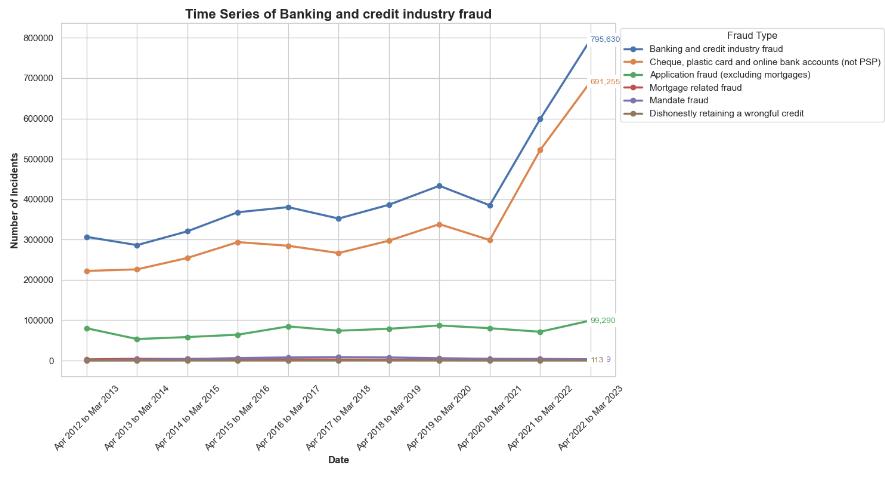
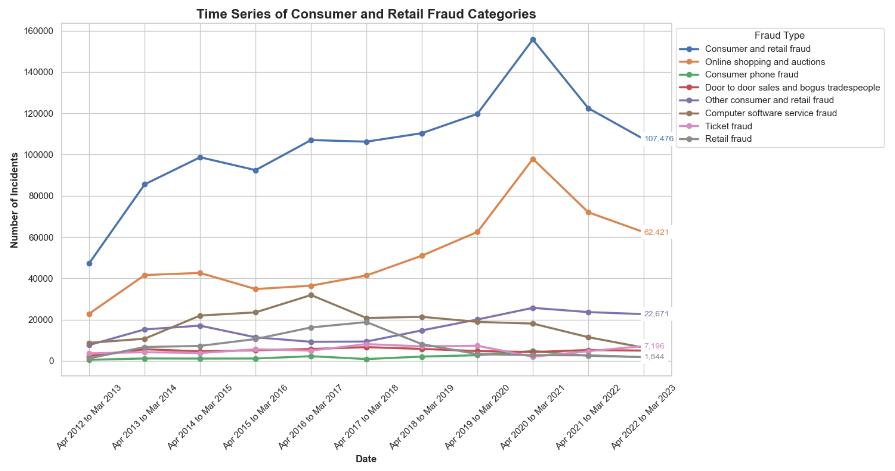
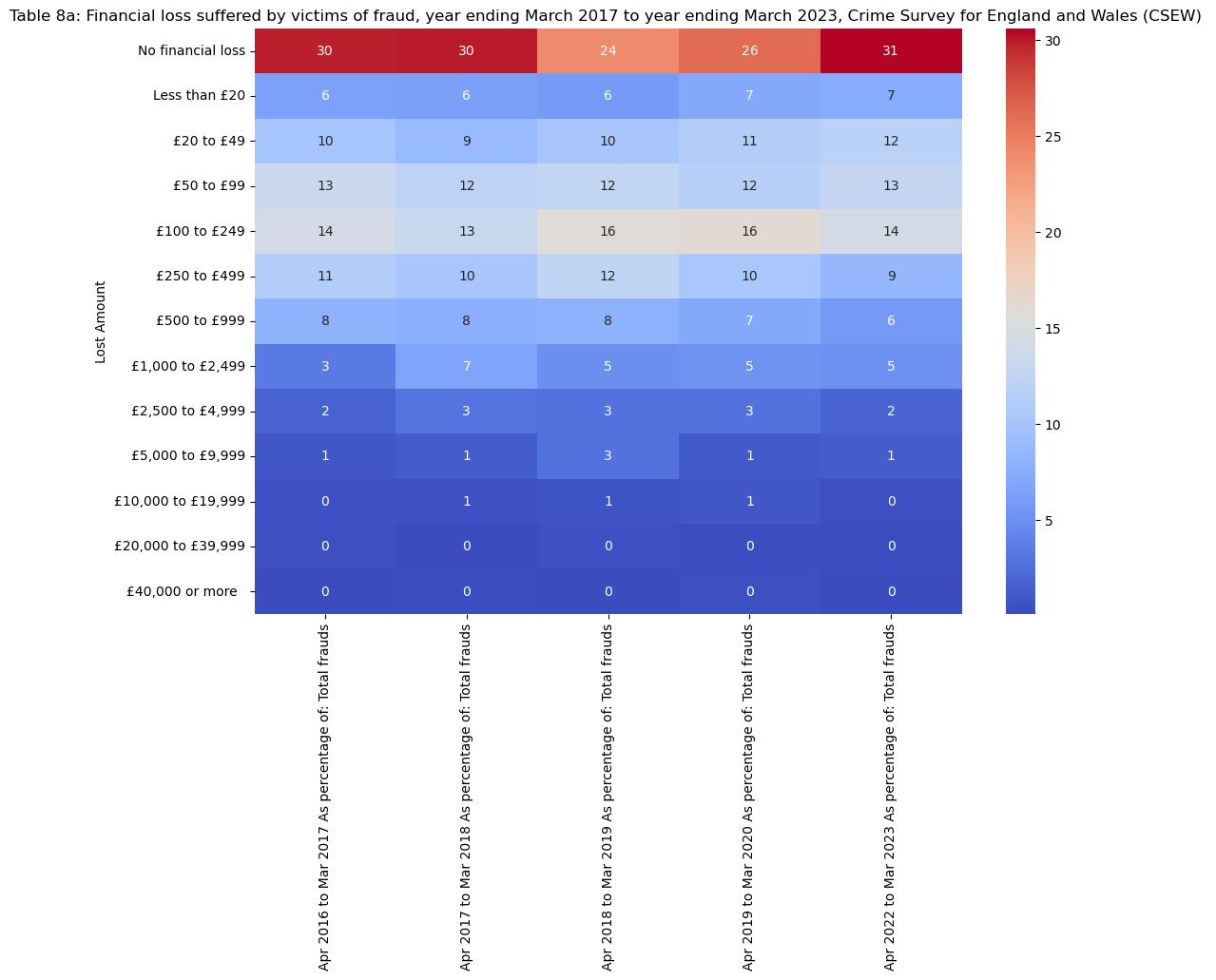
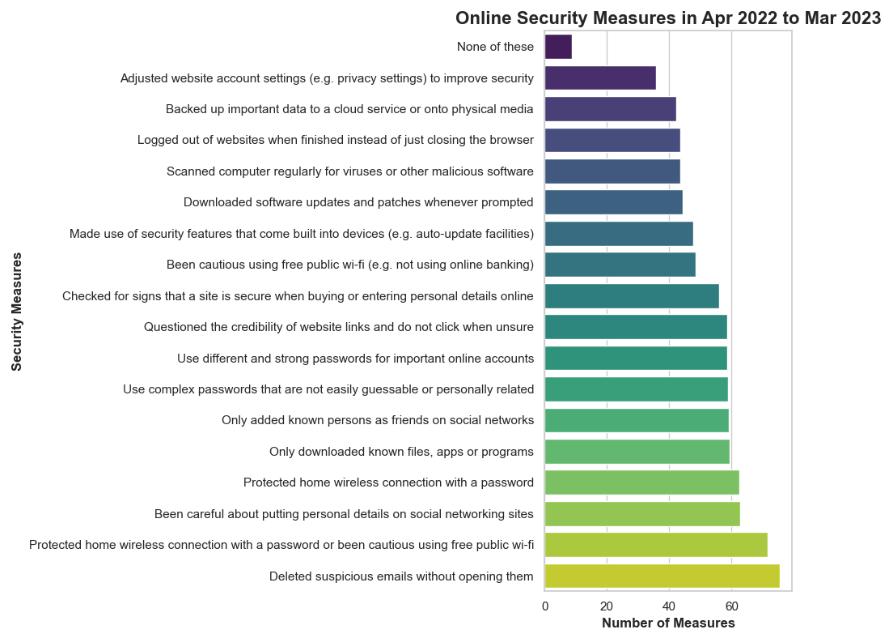
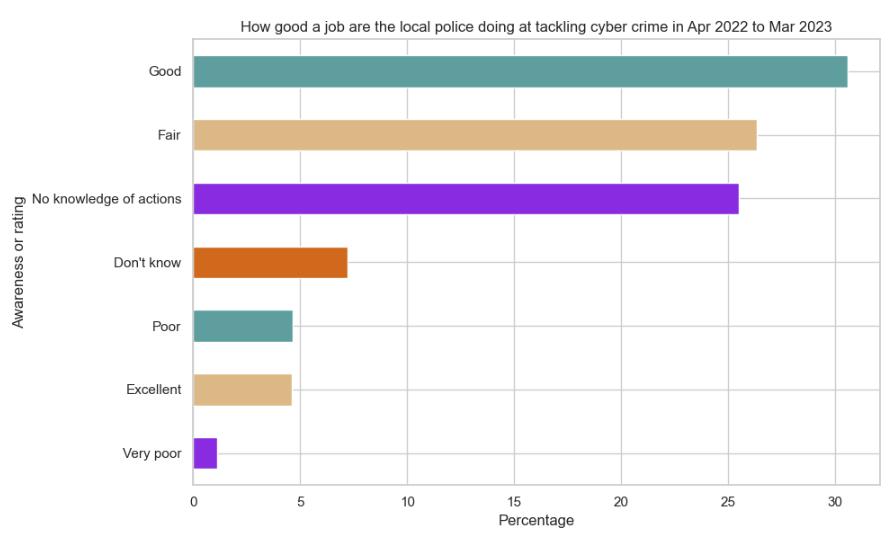